Personalized federal element learning method for data isomerism
A learning method and heterogeneous technology, applied in neural learning methods, machine learning, database updates, etc., can solve problems such as reducing the overall performance of personalized models, avoid negative transfer problems, and promote collaborative training
- Summary
- Abstract
- Description
- Claims
- Application Information
AI Technical Summary
Problems solved by technology
Method used
Image
Examples
Embodiment 1
[0067] like figure 1 The described personalized federated meta-learning method for data heterogeneity includes the following steps:
[0068] The first step is to determine the auto-encoder structure in the initialization phase of each client and the meta-model structure in the personalization phase;
[0069] Before each user's mobile device participates in federated learning, it first needs to download a unified auto-encoder and the model structure of the meta-model from the cloud server; the auto-encoder used in the initialization phase is a kind of neural network, which is generally used dimensionality reduction or feature learning, which is used here to represent the distribution of the user's local language data; the meta-model used in the personalization stage refers to a model under meta-learning, which can be trained by a small number of samples. A learning model adapted to new tasks. In order to predict the next word, a common language model is used here, such as LSTM...
Embodiment 2
[0110] like image 3 As shown, a total of 5 users participate in federated training, and they are divided into 2 groups in the cloud server. In any communication round, it is assumed that the grouping results of the previous round are {1, 2, 3} in the first group and {4, 5} in the second group. At this time, the cloud server selects users {1, 3, 5 } Participate in the federated training process, then users {1, 3 train each receive the meta model φ 1 , user {5} will receive the metamodel φ 2 .
[0111] For user 1, it is assumed that he has 100 local data, T=5 local updates are performed locally, and the batch size of random sampling for each update is , then after the local update is completed, the total sampled data size is min(2*5*5, 100)=50. Then calculate the distribution vector of this batch of sampled data together with the locally updated metamodel Upload to cloud server. Similarly, users {3, 5} also perform the above process.
[0112] For the cloud server, it ...
Embodiment 3
[0119] In an embodiment, the model structure of the auto-encoder may also be one of a convolutional auto-encoder and a cyclic auto-encoder,
PUM
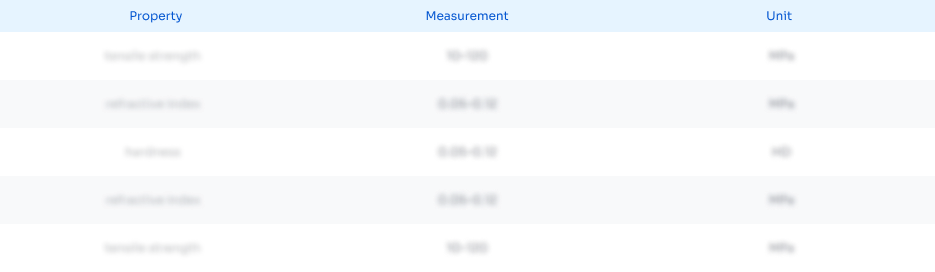
Abstract
Description
Claims
Application Information
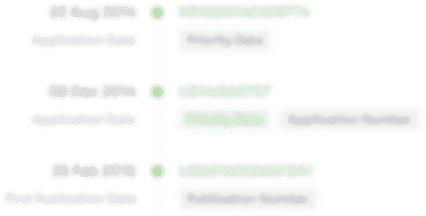
- R&D Engineer
- R&D Manager
- IP Professional
- Industry Leading Data Capabilities
- Powerful AI technology
- Patent DNA Extraction
Browse by: Latest US Patents, China's latest patents, Technical Efficacy Thesaurus, Application Domain, Technology Topic, Popular Technical Reports.
© 2024 PatSnap. All rights reserved.Legal|Privacy policy|Modern Slavery Act Transparency Statement|Sitemap|About US| Contact US: help@patsnap.com