End-to-end semi-supervised target detection method based on improved yolov5
A target detection and semi-supervised technology, applied in the field of target detection, can solve problems such as slow detection speed, failure to meet real-time requirements, complex process, etc., and achieve fast reasoning speed, good performance, and simple process
- Summary
- Abstract
- Description
- Claims
- Application Information
AI Technical Summary
Problems solved by technology
Method used
Image
Examples
Embodiment Construction
[0020] The technical solution of the present application will be described in detail below in conjunction with the accompanying drawings.
[0021] figure 1 It is a flowchart of the method described in this application, such as figure 1 As shown, the end-to-end semi-supervised target detection method based on improved yolov5 includes:
[0022] S1: Create a data set, and initialize the student model and the teacher model of the yolov5 network; wherein, the data set includes labeled data and unlabeled data, and the labeled data is divided into a training set and a test set.
[0023] S2: The yolov5 network loads the labeled data and unlabeled data respectively, and then performs strong data enhancement on the labeled data in the training set to obtain the first enhanced labeled data; respectively performs strong data enhancement and weak data enhancement on the unlabeled data, The first enhanced unlabeled data and the second enhanced unlabeled data are respectively obtained.
...
PUM
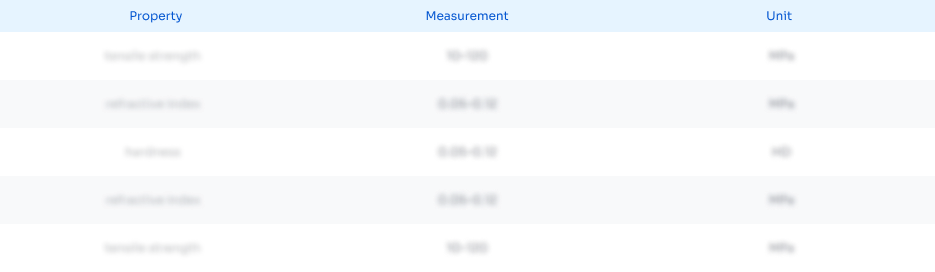
Abstract
Description
Claims
Application Information
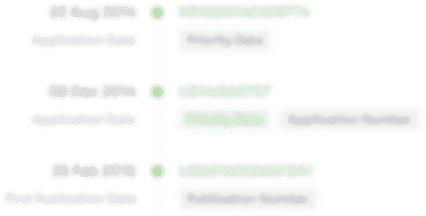
- R&D Engineer
- R&D Manager
- IP Professional
- Industry Leading Data Capabilities
- Powerful AI technology
- Patent DNA Extraction
Browse by: Latest US Patents, China's latest patents, Technical Efficacy Thesaurus, Application Domain, Technology Topic, Popular Technical Reports.
© 2024 PatSnap. All rights reserved.Legal|Privacy policy|Modern Slavery Act Transparency Statement|Sitemap|About US| Contact US: help@patsnap.com