Twin network change detection model based on deep learning
A twin network and change detection technology, applied in the field of change detection, can solve the problems of small amount of data, time-consuming, expensive, etc., and achieve the effect of improving model accuracy and improving the ability to extract differential features
- Summary
- Abstract
- Description
- Claims
- Application Information
AI Technical Summary
Problems solved by technology
Method used
Image
Examples
Embodiment Construction
[0032] The present invention will be described in further detail below in conjunction with the accompanying drawings and specific embodiments.
[0033] Such as figure 1 As shown, this embodiment discloses a Siamese network change detection model based on deep learning, including two modules of a generator and a discriminator, and the generator is a dual-branch computing model for obtaining difference images. We know that the generator includes two parts, the encoder and the decoder. In this scheme, ResNet18 is used as the model encoder to extract image feature information, and the twin network transformation is performed on the encoder. Two ResNet18 network parameters are shared for extraction respectively. A temporal feature map of a temporal phase. by the second branch convolutional network ( figure 1 middle ) and the upsampling convolutional network constitute the decoder, the second branch convolutional network is used to calculate the difference feature map accordin...
PUM
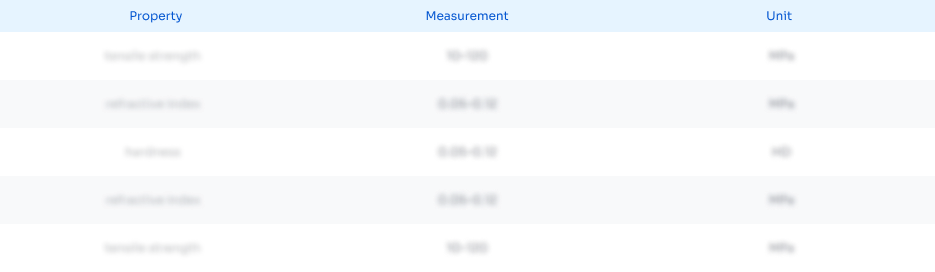
Abstract
Description
Claims
Application Information
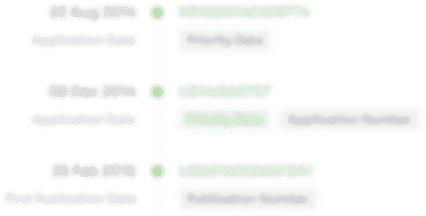
- R&D Engineer
- R&D Manager
- IP Professional
- Industry Leading Data Capabilities
- Powerful AI technology
- Patent DNA Extraction
Browse by: Latest US Patents, China's latest patents, Technical Efficacy Thesaurus, Application Domain, Technology Topic, Popular Technical Reports.
© 2024 PatSnap. All rights reserved.Legal|Privacy policy|Modern Slavery Act Transparency Statement|Sitemap|About US| Contact US: help@patsnap.com