Self-adaptive multi-mean two-step clustering method
A clustering method and mean clustering technology, applied in the field of data clustering, can solve the problems of sensitivity to outliers and high algorithm complexity, and achieve the effect of small influence of outliers, low complexity, and elimination of the influence of sensitive outliers.
- Summary
- Abstract
- Description
- Claims
- Application Information
AI Technical Summary
Problems solved by technology
Method used
Image
Examples
Embodiment
[0051] In the adaptive multi-means two-step clustering method of this embodiment, for the input data, the first step adopts the multi-means clustering algorithm based on chaotic quantum particle swarm optimization for preliminary clustering, and the second step adopts the adaptive hierarchical clustering algorithm for further clustering based on the clustering results of the first step to obtain the final clustering results; The flow chart of clustering method is as follows: Figure 1 The algorithm diagram is shown in Figure 2 The specific steps are as follows:
[0052] Step 1: multi mean clustering algorithm based on chaotic quantum particle swarm optimization; The flow chart of multi mean clustering algorithm is shown in Fig Figure 3 As shown in Figure 1.1, initialize clustering radius C r And allowable deviation C d , randomly select a data X i As the initial classification, the centroid of the classification is determined, and the clustering radius C is determined by the method...
PUM
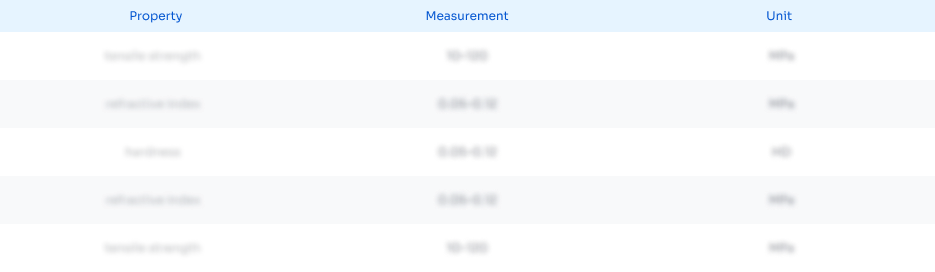
Abstract
Description
Claims
Application Information
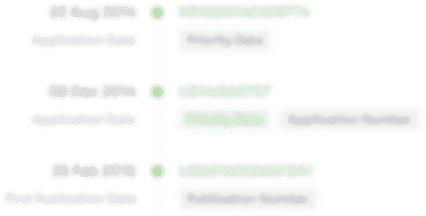
- R&D Engineer
- R&D Manager
- IP Professional
- Industry Leading Data Capabilities
- Powerful AI technology
- Patent DNA Extraction
Browse by: Latest US Patents, China's latest patents, Technical Efficacy Thesaurus, Application Domain, Technology Topic, Popular Technical Reports.
© 2024 PatSnap. All rights reserved.Legal|Privacy policy|Modern Slavery Act Transparency Statement|Sitemap|About US| Contact US: help@patsnap.com