SDN (Software Defined Network) flow control method based on fuzzy C-means and hybrid kernel least square support vector machine
A support vector machine and least squares technology, applied in computer parts, character and pattern recognition, instruments, etc., can solve the problem of not considering adaptability, and achieve the effect of improving prediction accuracy
- Summary
- Abstract
- Description
- Claims
- Application Information
AI Technical Summary
Problems solved by technology
Method used
Image
Examples
Embodiment Construction
[0054] The following will clearly and completely describe the technical solutions in the embodiments of the present invention with reference to the accompanying drawings in the embodiments of the present invention. Obviously, the described embodiments are only some, not all, embodiments of the present invention. Based on the embodiments of the present invention, all other embodiments obtained by persons of ordinary skill in the art without making creative efforts belong to the protection scope of the present invention.
[0055] A SDN network process control method based on fuzzy C-means and hybrid kernel least squares support vector machine, such as figure 1 As shown, the method includes: obtaining non-stationary SDN network traffic data, using discrete wavelet transform to convert non-stationary SDN network traffic data into stationary time series components; calculating the signal amplitude of stationary time series components, and using fast Fourier transform The signal amp...
PUM
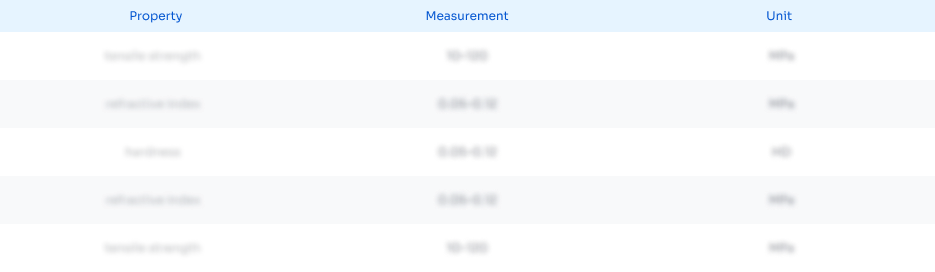
Abstract
Description
Claims
Application Information
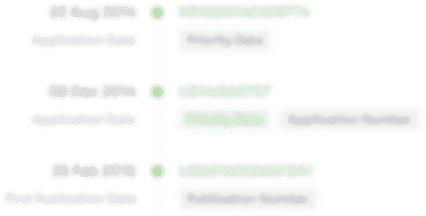
- R&D Engineer
- R&D Manager
- IP Professional
- Industry Leading Data Capabilities
- Powerful AI technology
- Patent DNA Extraction
Browse by: Latest US Patents, China's latest patents, Technical Efficacy Thesaurus, Application Domain, Technology Topic, Popular Technical Reports.
© 2024 PatSnap. All rights reserved.Legal|Privacy policy|Modern Slavery Act Transparency Statement|Sitemap|About US| Contact US: help@patsnap.com