Bearing residual life prediction method and device and medium
A life prediction and bearing technology, applied in bearing remaining life prediction, bearing remaining life prediction field based on convolution deep belief network-gated cycle unit, can solve the problems of unlabeled fault data, dimension disaster, low prediction accuracy, etc. , to achieve the effect of improving intelligence, improving stability, reducing time complexity and space complexity
- Summary
- Abstract
- Description
- Claims
- Application Information
AI Technical Summary
Problems solved by technology
Method used
Image
Examples
Embodiment Construction
[0028] In order to make the objectives, technical solutions and advantages of the present invention clearer, the present invention will be further described in detail below with reference to the embodiments and accompanying drawings. Here, the exemplary embodiments of the present invention and their descriptions are used to explain the present invention, but not to limit the present invention.
[0029] Here, it should also be noted that, in order to avoid obscuring the present invention due to unnecessary details, only the structures and / or processing steps closely related to the solution according to the present invention are shown in the drawings, and the related structures and / or processing steps are omitted. Other details not relevant to the invention.
[0030] It should be emphasized that the term "comprising / comprising" when used herein refers to the presence of a feature, element, step or component, but does not exclude the presence or addition of one or more other feat...
PUM
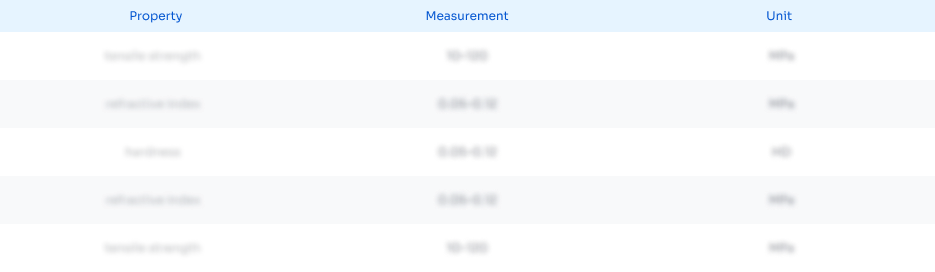
Abstract
Description
Claims
Application Information
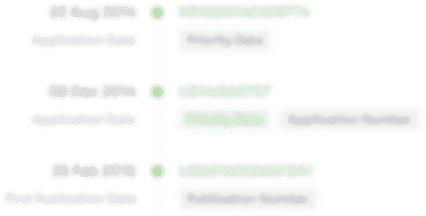
- R&D Engineer
- R&D Manager
- IP Professional
- Industry Leading Data Capabilities
- Powerful AI technology
- Patent DNA Extraction
Browse by: Latest US Patents, China's latest patents, Technical Efficacy Thesaurus, Application Domain, Technology Topic, Popular Technical Reports.
© 2024 PatSnap. All rights reserved.Legal|Privacy policy|Modern Slavery Act Transparency Statement|Sitemap|About US| Contact US: help@patsnap.com