Network energy consumption and throughput combined optimization routing method based on deep reinforcement learning
A technology of reinforcement learning and joint optimization, applied in the field of optical network communication, can solve problems such as increasing network overhead, and achieve the effect of reducing network energy consumption and high execution efficiency
- Summary
- Abstract
- Description
- Claims
- Application Information
AI Technical Summary
Problems solved by technology
Method used
Image
Examples
Embodiment
[0075] The invention models the business requirements, physical links and energy consumption of the data center network to generate training data, and performs training operations on the training data through a deep reinforcement learning (DRL) algorithm, so as to select the best route for business requirements. On the premise of satisfying service bearing, in order to maximize network throughput and reduce energy consumption. The specific approach is to first describe the routing scheduling of data center networks as a mixed integer nonlinear programming (MINLP) problem with two objectives, namely, maximizing network throughput and minimizing energy consumption; second, generating a large amount of training for deep reinforcement learning algorithms data, mainly including the current network state, decision-making behavior, reward and new network state; finally, convolutional neural network (CNN) and fully connected neural network (FC) are selected as the agent, and the traini...
PUM
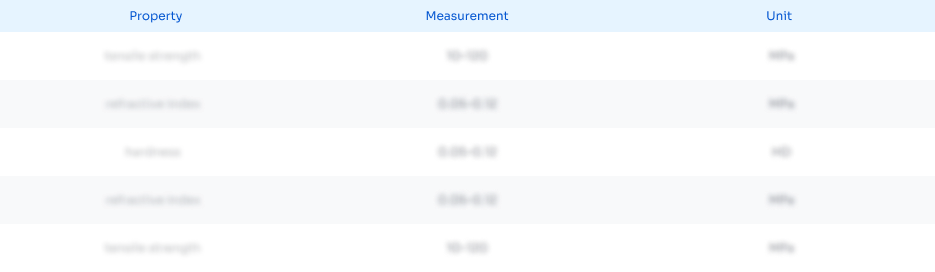
Abstract
Description
Claims
Application Information
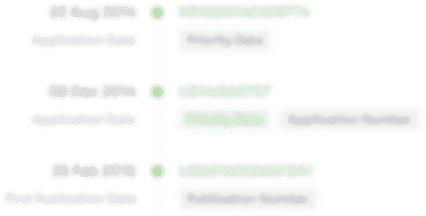
- R&D
- Intellectual Property
- Life Sciences
- Materials
- Tech Scout
- Unparalleled Data Quality
- Higher Quality Content
- 60% Fewer Hallucinations
Browse by: Latest US Patents, China's latest patents, Technical Efficacy Thesaurus, Application Domain, Technology Topic, Popular Technical Reports.
© 2025 PatSnap. All rights reserved.Legal|Privacy policy|Modern Slavery Act Transparency Statement|Sitemap|About US| Contact US: help@patsnap.com