Imprinted character recognition method and system based on deep learning
A recognition method and character technology, applied in the field of image recognition, can solve problems such as poor robustness and reduced generalization ability, and achieve high accuracy
- Summary
- Abstract
- Description
- Claims
- Application Information
AI Technical Summary
Problems solved by technology
Method used
Image
Examples
Embodiment 1
[0031] Embodiment 1 is an image character sequence recognition method, and the method includes the following steps: S1: preprocessing an original image to obtain pixel-level image data to be processed; S2: inputting the pixel-level image data to be processed into a convolutional neural network Perform feature extraction processing to obtain a feature map with a size of N*M*K, that is, a feature map of K channels with a size of N*M; S3: Perform a matrix transposition operation on the N*M*K feature map to obtain M*N *K feature map; tile the M*N*K feature image and convert it into a two-dimensional feature map of size M*(N*K); S4: The feature map of M*(N*K) is used The classification algorithm carries out the classification and discrimination of 1 categories, and obtains the sequence containing M classification results; in the M classification results, each discrimination result is a class in the 1 categories; S5: use the CTC algorithm to the sequence of the M classification resul...
Embodiment 2
[0042] In this embodiment, when the character sequence in the original image is an embossed digital character, a recognition model is obtained by training using the image character sequence recognition method, and the digital character is embossed. Its system block diagram refer to Figure 1 As shown, the system includes a training set acquisition module, a model training module, a to-be-recognized image acquisition module and an identification module.
[0043] (1) Training data acquisition module: use the camera to collect the imprinted character images, and try to avoid tilting when taking pictures. Scale the image to a fixed size (such as 72*288), and label the characters on the image to get the original dataset. The original dataset is divided into three datasets: training set, validation set and test set, which are used for model training, validation and testing respectively. In order to expand the dataset and increase the robustness of the model, it is necessary to per...
Embodiment 3
[0051] This embodiment is the process of using the image character sequence recognition method to train to obtain the recognition model:
[0052] 1. Training data acquisition module: use the camera to collect the imprinted character images, and try to avoid tilting when taking pictures.
[0053] 2. Scale the image to a fixed size (eg 72*288).
[0054] 3. Obtain 30,000 images through the above operations, and select 10,000 images as the test set. Annotate the remaining 20,000 images. First, encode according to the range of the font to be recognized, and then mark the characters on the image according to the encoding of each word. In particular, the code indicating blank should be added to the font code, but it is not necessary to mark when marking.
[0055] 4. Randomly shuffle and split into training set, validation set and test set. There are 15,000 images in the training set and 5,000 images in the validation set.
[0056] 5. Sharpen, blur and add noise to the training s...
PUM
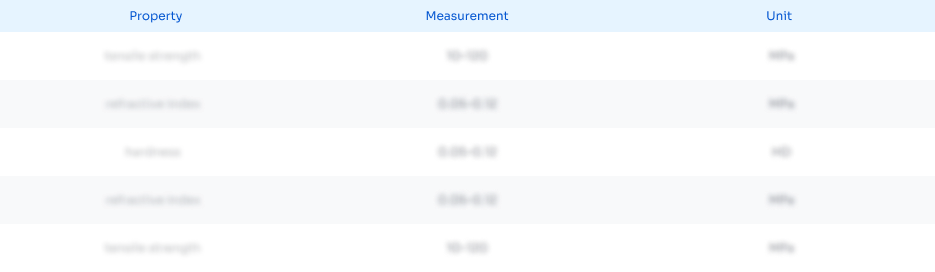
Abstract
Description
Claims
Application Information
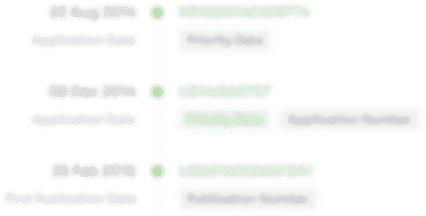
- R&D Engineer
- R&D Manager
- IP Professional
- Industry Leading Data Capabilities
- Powerful AI technology
- Patent DNA Extraction
Browse by: Latest US Patents, China's latest patents, Technical Efficacy Thesaurus, Application Domain, Technology Topic, Popular Technical Reports.
© 2024 PatSnap. All rights reserved.Legal|Privacy policy|Modern Slavery Act Transparency Statement|Sitemap|About US| Contact US: help@patsnap.com