Multi-mode and adversarial learning-based multi-task target detection and identification method and device
A target detection and multi-modal technology, applied in the field of deep learning target detection, can solve the problems of inability to generate models, fitting, low efficiency, etc., and achieve the effect of improving accuracy, improving robustness, fast and accurate detection and recognition
- Summary
- Abstract
- Description
- Claims
- Application Information
AI Technical Summary
Problems solved by technology
Method used
Image
Examples
Embodiment 1
[0095] The embodiment of the present invention proposes a target detection and recognition method based on multi-modal multi-task confrontation learning, such as figure 1 As shown, the method includes:
[0096] Step 1: Prepare the required image data set, normalize the data set images, and manually label the positions and types of objects in all data images; then use traditional data enhancement methods to expand the data set, and then use labelme software Label all RGB images with semantic information and generate a semantic map;
[0097] The details are as follows:
[0098] (1) The image training data set is collected through self-shooting and online search, and the images are required to contain different types of target objects.
[0099] (2) Normalize all image data, and convert the image into a standard image size of 256×256 pixels.
[0100] (3) Using the labelme software, the position of the target in the training sample image is closely surrounded by a rectangular f...
Embodiment 2
[0147] Based on the above method, an embodiment of the present invention also provides a multi-task target detection and recognition device based on multi-modality and confrontation learning, including:
[0148] Semantic map acquisition unit: through RGB image target annotation and preprocessing, and obtain the corresponding semantic map;
[0149] Recognition network construction unit: construct a multi-task recognition network model based on multi-modal and adversarial learning by using multi-modal feature fusion network, region proposal network and multi-task target detection network connected in sequence;
[0150] The multi-modal feature fusion network is formed by using two Resnet18 backbone CNN networks and then connecting the concat fusion network;
[0151] The region proposal network outputs random windows and proposal boxes;
[0152] The multi-tasks in the multi-task target detection network include three auxiliary tasks and one main task, wherein the main task is a t...
Embodiment 3
[0162] An example of the present invention also provides an electronic terminal, characterized in that: at least it includes:
[0163] one or more processors;
[0164] one or more memories;
[0165] The processor invokes the computer program stored in the memory to execute: the steps of the foregoing multi-task target detection and recognition method based on multi-modality and adversarial learning.
[0166] It should be understood that the specific implementation process refers to the related content of Embodiment 1.
[0167] The terminal also includes: a communication interface for communicating with external devices and performing data interactive transmission. For example, it communicates with the collection equipment of the operation information collection subsystem and the communication modules of other trains to obtain the real-time operation information of the train itself and its adjacent trains.
[0168] Among them, the memory may include high-speed RAM memory, an...
PUM
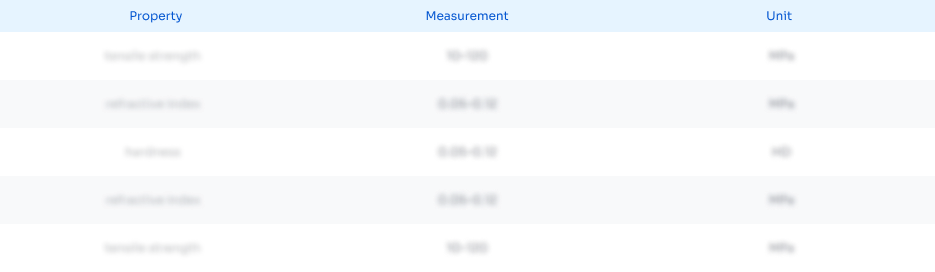
Abstract
Description
Claims
Application Information
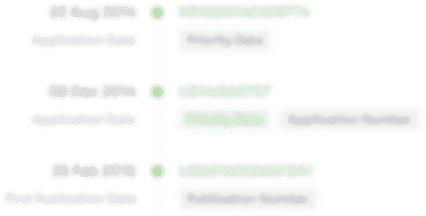
- R&D Engineer
- R&D Manager
- IP Professional
- Industry Leading Data Capabilities
- Powerful AI technology
- Patent DNA Extraction
Browse by: Latest US Patents, China's latest patents, Technical Efficacy Thesaurus, Application Domain, Technology Topic, Popular Technical Reports.
© 2024 PatSnap. All rights reserved.Legal|Privacy policy|Modern Slavery Act Transparency Statement|Sitemap|About US| Contact US: help@patsnap.com