Drought disaster weather prediction method based on semi-supervised ensemble learning
An integrated learning and weather prediction technology, applied in climate sustainability, instrumentation, design optimization/simulation, etc., can solve problems such as low confidence level, poor generalization performance, and slow training speed, so as to improve training efficiency and improve The effect on generalization performance
- Summary
- Abstract
- Description
- Claims
- Application Information
AI Technical Summary
Problems solved by technology
Method used
Image
Examples
Embodiment Construction
[0035] A method for forecasting drought disaster weather based on semi-supervised ensemble learning, characterized by comprising the following steps:
[0036]Step (1): establish a data sample set for predicting drought disaster weather, specifically including WS10M_MIN minimum wind speed of 10 meters (m / s), QV2M 2 meters of relative humidity (g / kg), T2M_RANGE 2 meters of temperature range ( ℃), WS10M wind speed 10 meters (m / s), T2M 2 meters temperature (℃), WS50M_MIN 50 meters minimum wind speed (m / s), T2M_MAX 2 meters maximum temperature (℃), WS50M 50 meters wind speed (m / s), TS Earth skin temperature (°C), WS50M_RANGE wind speed range at 50 meters (m / s), WS50M_MAX maximum wind speed at 50 meters (m / s), WS10M_MAX maximum wind speed at 10 meters (m / s) ), WS10M_RANGE wind speed range at 10 meters (m / s), PS surface pressure (kPa), T2MDEW 2 meters dew point / freezing point (℃), T2M_MIN 2 meters minimum temperature (℃), T2MWET 2 meters wet bulb temperature (℃) ), PRECTOT precipita...
PUM
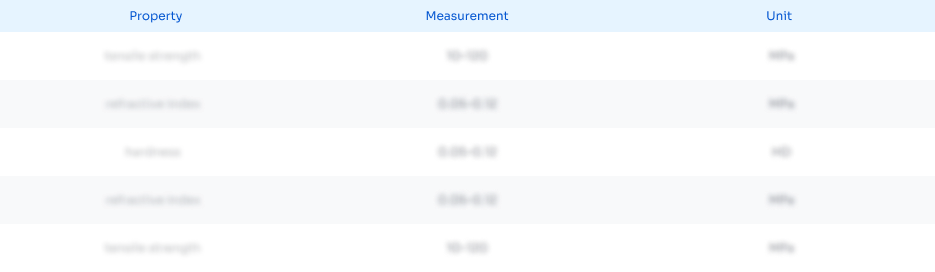
Abstract
Description
Claims
Application Information
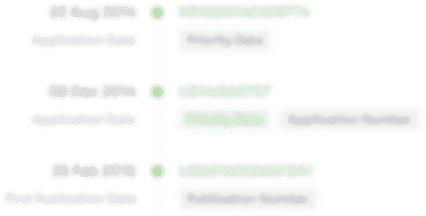
- R&D
- Intellectual Property
- Life Sciences
- Materials
- Tech Scout
- Unparalleled Data Quality
- Higher Quality Content
- 60% Fewer Hallucinations
Browse by: Latest US Patents, China's latest patents, Technical Efficacy Thesaurus, Application Domain, Technology Topic, Popular Technical Reports.
© 2025 PatSnap. All rights reserved.Legal|Privacy policy|Modern Slavery Act Transparency Statement|Sitemap|About US| Contact US: help@patsnap.com