Cognitive tracking method fusing knowledge association path
A path and knowledge technology, applied in the field of cognitive tracking that integrates knowledge association paths, can solve problems such as inaccurate prediction results, path diagrams that do not consider knowledge point associations, and knowledge points predict students' answer performance, etc., to achieve the effect of improving performance
- Summary
- Abstract
- Description
- Claims
- Application Information
AI Technical Summary
Problems solved by technology
Method used
Image
Examples
Embodiment Construction
[0043] In this embodiment, a cognitive tracking method integrating knowledge association paths, such as figure 1 As shown, the steps are as follows:
[0044] Step 1. According to the corresponding relationship between exercises and knowledge points in the data set, the acquisition dimension is n q ×n s The question-knowledge point correlation matrix QS, in which all exercises included in QS are recorded as q i represents the ith exercise, n q is the total number of exercises; all knowledge points included in QS are recorded as s j represents the jth knowledge point, n s is the total number of knowledge points; if the i-th exercise q i with the jth knowledge point s j associated, then let the element QS of the i-th row and the j-th column in the correlation matrix QS i,j =1;
[0045] For example, all exercises q in the dataset 1 , q 2 , q 3 and all knowledge points 1 , s 2 , s 3 The corresponding relationship is:
[0046] q 1 Corresponding knowledge point s ...
PUM
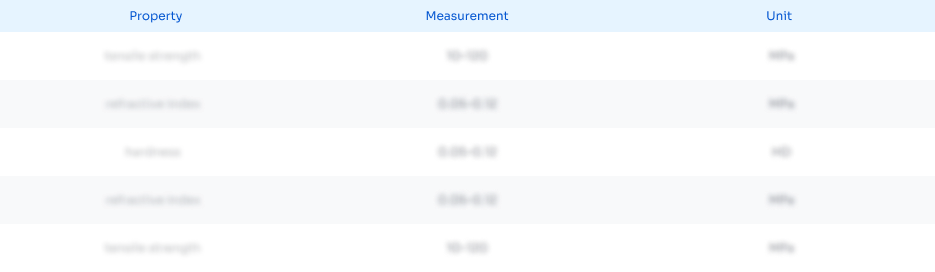
Abstract
Description
Claims
Application Information
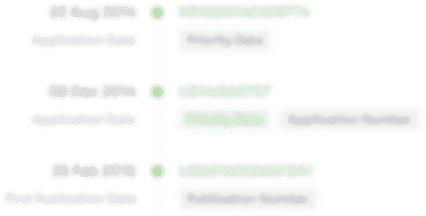
- R&D Engineer
- R&D Manager
- IP Professional
- Industry Leading Data Capabilities
- Powerful AI technology
- Patent DNA Extraction
Browse by: Latest US Patents, China's latest patents, Technical Efficacy Thesaurus, Application Domain, Technology Topic, Popular Technical Reports.
© 2024 PatSnap. All rights reserved.Legal|Privacy policy|Modern Slavery Act Transparency Statement|Sitemap|About US| Contact US: help@patsnap.com