Human motion date recognizing method based on integrated Hidden Markov model leaning method
A technology that integrates Hidden Markov and Hidden Markov, applied in character and pattern recognition, instruments, computer parts, etc. The effect of eliminating data redundancy and reducing the amount of calculation
- Summary
- Abstract
- Description
- Claims
- Application Information
AI Technical Summary
Problems solved by technology
Method used
Image
Examples
Embodiment 1
[0089] The training samples include 150 walking motions of various types, with Figure 4 The comparison of the accuracy of walking motion recognition using two learning methods of weak hidden Markov model and integrated hidden Markov model is given. The concrete steps that the method of the present invention is described in detail below this example implementation are as follows:
[0090] (1) Extract all the two-dimensional geometric features of the walking motion by the method described in step 1: extract a two-dimensional geometric feature to express that a right toe is located in front of the plane composed of the left ankle, left hip and root node in the walking motion fixed posture. we define , 1≤i≤4 are four three-dimensional points, where 1 ,p 2 ,p 3 >Indicates the datum plane determined by the first three points, and the orientation depends on the order of the three points. Then we define as follows:
[0091]
[0092] Through the above definition, we define a...
Embodiment 2
[0124] The training samples include 110 boxing sports of various types, with Figure 5 The comparison of boxing motion recognition accuracy is given by two learning methods of weak hidden Markov model and integrated hidden Markov model. The concrete steps that the method of the present invention is described in detail below this example implementation are as follows:
[0125] (1) Extract a two-dimensional geometric feature using the method described in step 1 to express a fixed posture in which a right hand is located in front of the plane formed by the left shoulder, left hip and root node in boxing. we define p i ∈ R 3 , 1≤i≤4 are four three-dimensional points, where 1 ,p 2 ,p 3 >Indicates the datum plane determined by the first three points, and the orientation depends on the order of the three points. Then we define as follows:
[0126]
[0127] Through the above definition, we define a feature function for any four adjacent joint points as follows:
[0128] ...
PUM
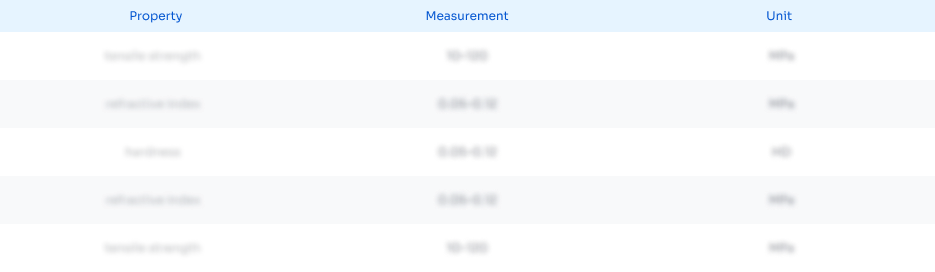
Abstract
Description
Claims
Application Information
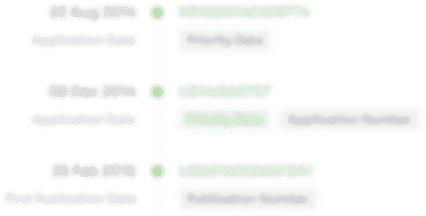
- R&D Engineer
- R&D Manager
- IP Professional
- Industry Leading Data Capabilities
- Powerful AI technology
- Patent DNA Extraction
Browse by: Latest US Patents, China's latest patents, Technical Efficacy Thesaurus, Application Domain, Technology Topic, Popular Technical Reports.
© 2024 PatSnap. All rights reserved.Legal|Privacy policy|Modern Slavery Act Transparency Statement|Sitemap|About US| Contact US: help@patsnap.com