Deep space autonomous navigation method based on observability degree analysis
A technology of autonomous navigation and autonomous navigation system, which is applied in the field of aerospace and aviation, can solve the problems of lower utilization rate of observation information, lower adaptive ability and reliability of autonomous navigation system, etc., to improve adaptive ability and reliability, and improve utilization rate Effect
- Summary
- Abstract
- Description
- Claims
- Application Information
AI Technical Summary
Problems solved by technology
Method used
Image
Examples
specific Embodiment approach 1
[0013] Specific implementation mode one: combine figure 1 Describe this embodiment, a deep space autonomous navigation method based on observability analysis described in this embodiment, the specific steps are:
[0014] Step 1: Establish the state model of the deep space autonomous navigation system of the deep space probe based on the dynamic model of the Earth-Moon transfer orbit;
[0015] Step 2: Establish the earth-center line-of-sight vector observation model and the moon-center line-of-sight vector observation model of the deep space probe based on the earth-moon transfer orbit;
[0016] Step 3: Analyze the state model of the autonomous navigation system in step 1 and the geocentric line-of-sight vector observation model and moon-centered line-of-sight vector observation model obtained in step 2 by using the nonlinear system observability analysis method; obtain the geocentric line-of-sight vector observation model The observability of the autonomous navigation system ...
specific Embodiment approach 2
[0021] Specific embodiment two: the difference between this embodiment and the deep space autonomous navigation method based on observability analysis described in embodiment one is that the state model of the deep space autonomous navigation system described in step one is:
[0022]
[0023] In the formula, r=[xyz] T is the position of the detector in the inertial system, v=[v x v y v z ] T is the speed of the detector in the inertial system, X=[r T v T ] T is the system state variable; μ is the gravitational constant of the earth, J 2 is the gravitational coefficient perturbed by the shape of the earth, R c is the mean equatorial radius of the earth; where x m ,y m ,z m is the position component of the moon in the inertial system, r m is the radius of the moon in the inertial system; r sm is the radius of the moon relative to the probe; w is the error of the system model, and the state model of the above-mentioned deep space autonomous navigation system is als...
specific Embodiment approach 3
[0025] Specific implementation mode three: combination figure 2 Describe this embodiment. The difference between this embodiment and the deep-space autonomous navigation method based on observability analysis described in Embodiment 1 lies in the observation model of the earth-centered line-of-sight vector and the moon-centered line-of-sight vector observation described in step 2. model, whose expression is as follows:
[0026] The geocentric line-of-sight vector observation model is
[0027]
[0028] where Θ e is the altitude angle in the direction of the center of the earth, Φ e is the geocentric azimuth;
[0029] The lunar center line of sight vector observation model is
[0030]
[0031] where Θ m is the altitude angle in the direction of the moon center, Φ m is the azimuth of the lunar center.
[0032] In this embodiment, the earth-centered line-of-sight vector and the moon-centered line-of-sight vector are measured observation equations, and the attitude co...
PUM
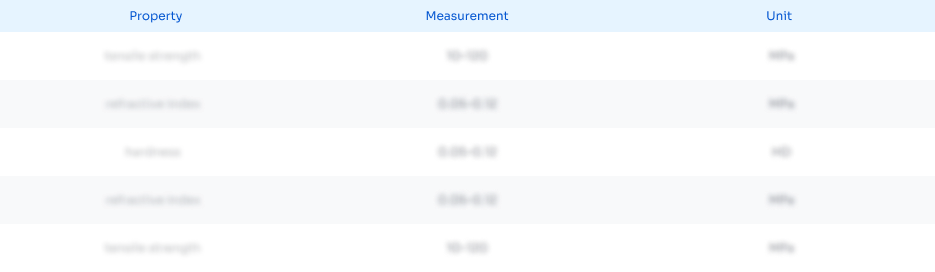
Abstract
Description
Claims
Application Information
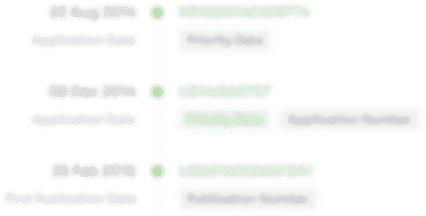
- R&D
- Intellectual Property
- Life Sciences
- Materials
- Tech Scout
- Unparalleled Data Quality
- Higher Quality Content
- 60% Fewer Hallucinations
Browse by: Latest US Patents, China's latest patents, Technical Efficacy Thesaurus, Application Domain, Technology Topic, Popular Technical Reports.
© 2025 PatSnap. All rights reserved.Legal|Privacy policy|Modern Slavery Act Transparency Statement|Sitemap|About US| Contact US: help@patsnap.com