Multi-kernel support vector machine classification method for remote sensing images
A technology of support vector machine and classification method, which is applied in the field of support vector machine classification to achieve the effects of improving classification accuracy and reliability, improving accuracy, and improving classification accuracy
- Summary
- Abstract
- Description
- Claims
- Application Information
AI Technical Summary
Problems solved by technology
Method used
Image
Examples
Embodiment 1
[0015] Example 1: Using OMIS in Changping District, Beijing Hyperspectral image, with a size of 512 rows, 512 columns, and 64 bands. In the comparison of classification methods of multi-core support vector machines, spectral information and wavelet texture are combined first, then the first 4 components of PCA transform and wavelet texture are used for classification, and finally one-dimensional wavelet texture and two-dimensional wavelet texture are combined for classification. The classification results and Kappa coefficients are shown in Table 1.
[0016] Specific implementation steps:
[0017] (1) Preprocess the original multispectral or hyperspectral remote sensing image data, remove noise bands, and then determine the classification system and select training samples.
[0018] (2) Perform principal component transformation on the data set obtained in step (1), select the first four components as spectral features to participate in classification, and use Represents ...
Embodiment 2
[0031] Embodiment 2: The experimental data is the data of the University of Pavia in Italy acquired by the ROSIS sensor in 2003. Experimental data 1 is the same as experimental data 2. First, single-core SVM classification is performed, and then multi-core SVM classification is performed. The classification accuracy is shown in Table 3 and Table 4.
[0032] Table 3 Single-core SVM classification accuracy
[0033] .
[0034] Table 4 Multi-core SVM classification accuracy
[0035]
[0036] It can be seen that the result of test 2 is the same as that of test 1, and the multi-core support vector machine method using the first four components of principal component transformation and one-dimensional wavelet texture features can obtain the highest precision, which fully demonstrates the reliability and effectiveness of the present invention.
PUM
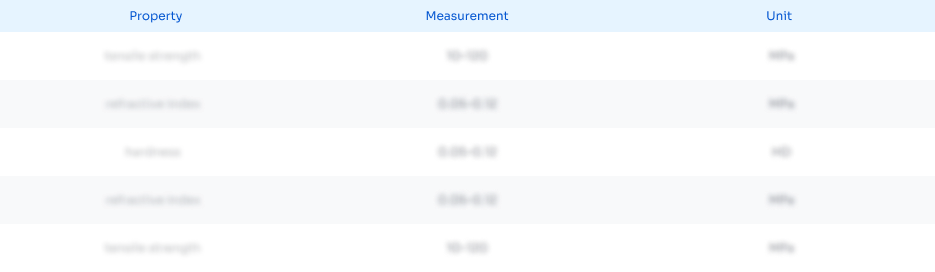
Abstract
Description
Claims
Application Information
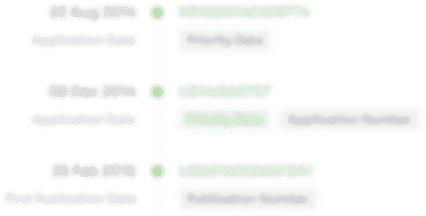
- R&D Engineer
- R&D Manager
- IP Professional
- Industry Leading Data Capabilities
- Powerful AI technology
- Patent DNA Extraction
Browse by: Latest US Patents, China's latest patents, Technical Efficacy Thesaurus, Application Domain, Technology Topic, Popular Technical Reports.
© 2024 PatSnap. All rights reserved.Legal|Privacy policy|Modern Slavery Act Transparency Statement|Sitemap|About US| Contact US: help@patsnap.com