Image super-resolution reconstruction method based on multitask KSVD (K singular value decomposition) dictionary learning
A technology of super-resolution reconstruction and dictionary learning, applied in the field of image processing, can solve the problems of long image reconstruction time, degraded image quality, and deviation of image reconstruction effect, so as to shorten the image reconstruction time and improve the reconstruction efficiency. , the effect of reducing the number of
- Summary
- Abstract
- Description
- Claims
- Application Information
AI Technical Summary
Problems solved by technology
Method used
Image
Examples
Embodiment Construction
[0036] Refer to attached figure 1 , the concrete steps of the present invention are as follows:
[0037] Step 1. Preprocess and classify training images
[0038] 1a) Input training low-resolution and high-resolution image pairs, and filter them to extract features. The filter bank expression used is f 1 =[-1,0,1], f 3 =[1,0,-2,0,1], The training images used are as image 3 , Figure 4 , Figure 5 and Image 6 not;
[0039] 1b) from image 3 , Figure 4 , Figure 5 and Image 6 Randomly extract 100,000 pairs of small image blocks, construct a matrix M, use the K-means algorithm to divide the small image blocks in the matrix M into 5 categories, but not limited to 5 categories, and obtain 5 pairs of initial high-resolution dictionary H 1 , H 2 , H 3 , H 4 , H 5 and a low-resolution dictionary L 1 , L 2 , L 3 , L 4 , L 5 , and 5 cluster centers C 1 , C 2 , C 3 , C 4 , C 5 , but not limited to 5 pairs of initial dictionaries and 5 cluster centers.
[...
PUM
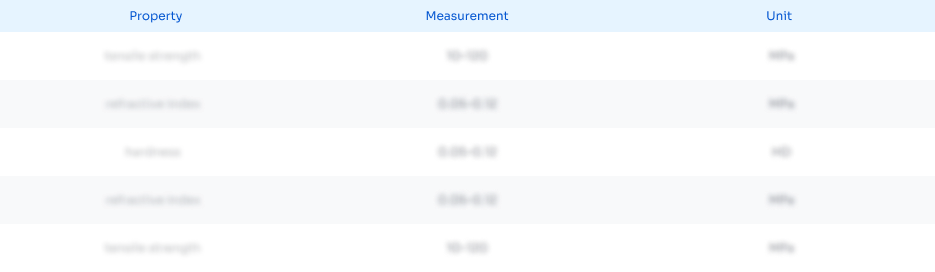
Abstract
Description
Claims
Application Information
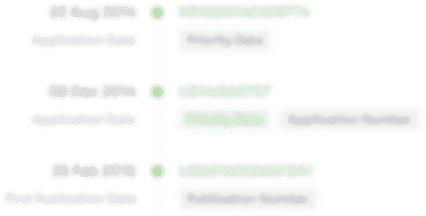
- R&D Engineer
- R&D Manager
- IP Professional
- Industry Leading Data Capabilities
- Powerful AI technology
- Patent DNA Extraction
Browse by: Latest US Patents, China's latest patents, Technical Efficacy Thesaurus, Application Domain, Technology Topic.
© 2024 PatSnap. All rights reserved.Legal|Privacy policy|Modern Slavery Act Transparency Statement|Sitemap