Rapid keyword detection method based on quantile self-adaption cutting
A technology of quantiles and keywords, applied in the field of fast adaptive clipping of local paths, can solve the problems of low system efficiency and inability to effectively clip local paths to the greatest extent, so as to improve detection efficiency, reduce scale, and increase recognition speed Effect
- Summary
- Abstract
- Description
- Claims
- Application Information
AI Technical Summary
Problems solved by technology
Method used
Image
Examples
specific Embodiment approach 1
[0029] Embodiment 1: This embodiment is a fast keyword detection method based on quantile adaptive clipping, which is realized through the following steps:
[0030] Step 1, input the speech signal to be detected, carry out preprocessing to the input speech signal to be detected, feature extraction obtains speech feature vector sequence X={x 1 , x 2 ,...x S}, where S represents a natural number;
[0031] Step 2, according to the Viterbi decoding algorithm, the speech feature vector sequence is decoded on the pre-defined recognition network;
[0032] Step 3. For any time t, all local paths are extended forward once to obtain the corresponding active models on the corresponding local paths, and the state of each active model is calculated at the same time to generate x t , and summing the states of each active model yields x t The probability of the corresponding local path probability score, where, x t ∈X, 1≤t≤S, t is an integer;
[0033] Step 4. Carry out quantile-based s...
specific Embodiment approach 2
[0040] Embodiment 2: The difference between this embodiment and Embodiment 1 is that in step 4, the quantile-based state layer local path clipping is performed, as follows:
[0041] Step 1. Set the percentage α and the weighting factor λ of the local path required to be reserved at time t, where the value of α is 0<α<1, and the value of λ is 1<λ<3;
[0042] Step 2. Save all local path probability scores at time t (that is, the corresponding local path probability scores obtained in step 3) into the array score[1...N], assuming that there are N local paths at time t;
[0043] Step 3. Find the number S with the largest N×α in score[1...N] according to the binary search algorithm α , that is, the upper α quantile;
[0044] Step 4. Set the beam width clipped at time t as beam(t)=λ×(S max -S α ) (1<λ<3);
[0045] Step 5, set the clipping threshold at time t as thresh(t)=S max -beam(t), where S max is the maximum number in the array score[1...N];
[0046] Step 6. Traverse eac...
specific Embodiment approach 3
[0049] Specific embodiment three: the difference between this embodiment and specific embodiment one or two is that the process of feature extraction in step one to obtain the feature vector sequence is: the speaker signal s(n) (i.e. the speech signal to be detected) is sampled and quantized and Pre-emphasis processing, assuming that the speaker signal is short-term stable, so the speaker signal can be divided into frames. The specific frame division method is realized by using a movable finite length window for weighting. The weighted voice signal the s w (n) calculate Mel cepstrum coefficient (MFCC parameter), thereby obtain feature vector sequence X={x 1 , x 2 ,...,x s}. Other steps and parameters are the same as those in Embodiment 1 or Embodiment 2.
PUM
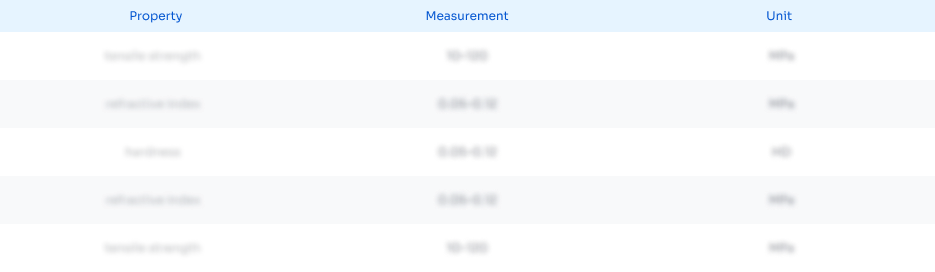
Abstract
Description
Claims
Application Information
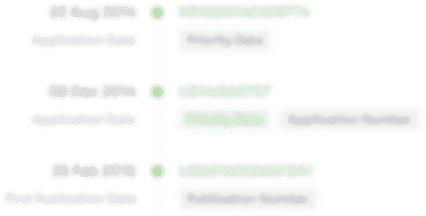
- R&D Engineer
- R&D Manager
- IP Professional
- Industry Leading Data Capabilities
- Powerful AI technology
- Patent DNA Extraction
Browse by: Latest US Patents, China's latest patents, Technical Efficacy Thesaurus, Application Domain, Technology Topic, Popular Technical Reports.
© 2024 PatSnap. All rights reserved.Legal|Privacy policy|Modern Slavery Act Transparency Statement|Sitemap|About US| Contact US: help@patsnap.com