Data-driven lithium ion battery cycle life prediction method based on AR (Autoregressive) model and RPF (Regularized Particle Filtering) algorithm
A lithium-ion battery, AR model technology, applied in the direction of measuring electricity, measuring devices, measuring electrical variables, etc., can solve the problems of complex forecasting method modeling and difficult parameter identification, and achieve trend forecasting, a good method framework, and improve accuracy. Effect
- Summary
- Abstract
- Description
- Claims
- Application Information
AI Technical Summary
Problems solved by technology
Method used
Image
Examples
specific Embodiment approach 1
[0029] Specific implementation mode one: the data-driven lithium-ion battery cycle life prediction method based on the AR model and the RPF algorithm described in this implementation mode is:
[0030] 1) Monitor various physical parameters of the lithium-ion battery to be predicted, and obtain monitoring data;
[0031] 2) Use the RPF algorithm to track the state of the battery capacity data of the monitoring data, and determine the unknown parameter β in the empirical model of the RPF particle battery degradation battery 1 and beta 2 ;
[0032] 3) Initialize and set the starting point of prediction, the number of particles N, and the process noise W in the regularized particle filter model k The covariance R of , the observation noise V in the regularized particle filter model k The covariance Q of , the threshold U of the end of battery life;
[0033] 4) Determine the training length Length according to the prediction starting point, and use the battery capacity historica...
specific Embodiment approach 2
[0044] Embodiment 2: This embodiment is a further limitation of the data-driven lithium-ion battery cycle life prediction method based on the AR model and the RPF algorithm described in Embodiment 1. In Embodiment 1, the specific process of step 1 is as follows :
specific Embodiment approach 3
[0045] Specific embodiment three: The described RPF particle battery degraded battery empirical model is:
[0046] C k+1 = η C C k +β 1 exp(-β 2 / Δt k ) (1)
[0047] Among them, C k Indicates the charge capacity of the kth charge-discharge cycle, Δt k Indicates the rest time from the kth cycle to the k+1th cycle, β 1 and beta 2 is the parameter to be determined.
[0048] PF-based battery cycle life prediction method such as figure 2 shown. The entire prediction framework consists of four parts: sensor data acquisition and processing, data feature extraction, particle filter state tracking, long-term prediction of battery capacity, and cycle life calculation.
[0049] The PF algorithm in the prediction needs to establish a state transition equation and an observation equation based on the battery degradation process model, but in actual working conditions, it is difficult to establish an accurate physical model of the battery degradation process based on the ele...
PUM
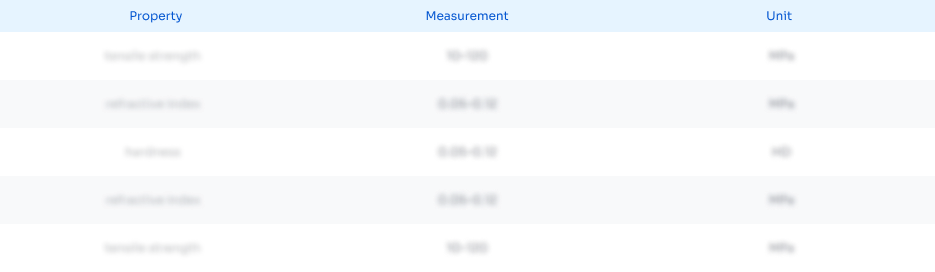
Abstract
Description
Claims
Application Information
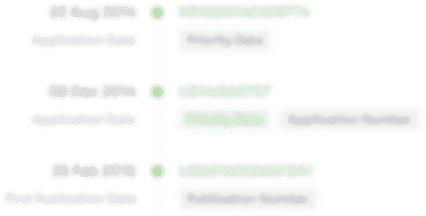
- R&D Engineer
- R&D Manager
- IP Professional
- Industry Leading Data Capabilities
- Powerful AI technology
- Patent DNA Extraction
Browse by: Latest US Patents, China's latest patents, Technical Efficacy Thesaurus, Application Domain, Technology Topic.
© 2024 PatSnap. All rights reserved.Legal|Privacy policy|Modern Slavery Act Transparency Statement|Sitemap