Compressed sensing signal reconstruction method
A compressed sensing and signal reconstruction technology, applied in the field of signal processing, can solve the problems of low reconstruction accuracy, slow algorithm convergence speed, and low reconstruction accuracy, and achieve the effect of high accuracy and speeding up the convergence speed.
- Summary
- Abstract
- Description
- Claims
- Application Information
AI Technical Summary
Problems solved by technology
Method used
Image
Examples
Embodiment Construction
[0027] The technical scheme of the present invention is described in detail below in conjunction with accompanying drawing:
[0028] In order to facilitate the public to understand the technical solution of the present invention, a brief introduction to the basic knowledge of compressed sensing is given below.
[0029] A key foundation of modern signal processing is Shannon's sampling theory: the number of discrete samples required for a signal to be reconstructed without distortion is determined by its bandwidth. But Shannon sampling theorem is a sufficient but not necessary condition for signal reconstruction. As a new sampling theory, compressed sensing can obtain discrete samples of the signal at a rate much smaller than that of Nyquist, ensuring the distortion-free reconstruction of the signal. The core idea of compressive sensing theory mainly includes two points. The first is the sparse structure of the signal. The traditional Shannon signal representation method o...
PUM
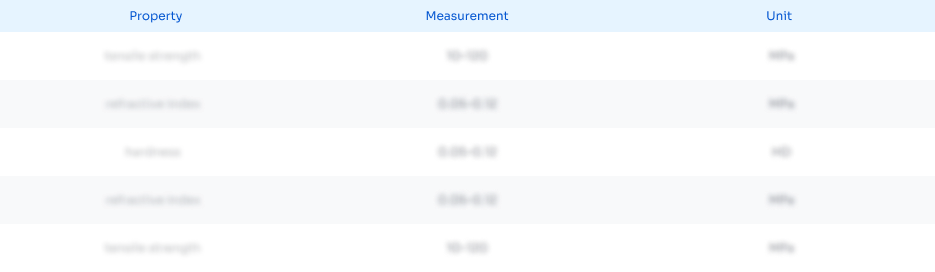
Abstract
Description
Claims
Application Information
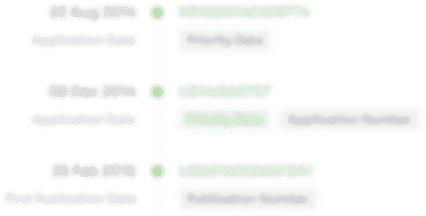
- R&D Engineer
- R&D Manager
- IP Professional
- Industry Leading Data Capabilities
- Powerful AI technology
- Patent DNA Extraction
Browse by: Latest US Patents, China's latest patents, Technical Efficacy Thesaurus, Application Domain, Technology Topic.
© 2024 PatSnap. All rights reserved.Legal|Privacy policy|Modern Slavery Act Transparency Statement|Sitemap