Intelligent transformer fault diagnostic method based on RBF (radial basis function) neural network
A transformer fault and neural network technology, applied in neural learning methods, biological neural network models, instruments, etc., can solve the problems that the three ratios do not include and reflect transformers, easy misjudgment, transformer misdiagnosis, etc., to improve reasoning ability and diagnostic accuracy, expanding the scope of diagnosis, and improving the effect of accuracy
- Summary
- Abstract
- Description
- Claims
- Application Information
AI Technical Summary
Problems solved by technology
Method used
Image
Examples
Embodiment Construction
[0030] The present invention proposes an intelligent method for transformer fault diagnosis based on RBF neural network, such as figure 1 As shown, it specifically includes the following steps:
[0031] (1), use the IEC three-ratio method to draw the three-ratio values of five gases: C 2 h 2 / C 2 h 4 、CH 4 / H 2 、C 2 h 4 / C 2 h 6 as training sample data;
[0032] (2), utilize membership function to carry out fuzzy processing with three ratios;
[0033] (3), code the fault type;
[0034] (4), according to the corresponding relationship between the fuzzy three-ratio data and the fault type as sample data, train the RBF neural network until the RBF network meets the accuracy requirements and then enters the next step;
[0035] (5), input the sample to be tested after fuzzy processing;
[0036] (6) Output the diagnosis result.
[0037] Radial basis network neural network (RBF network for short) described in the present invention is a three-layer feed-forward network...
PUM
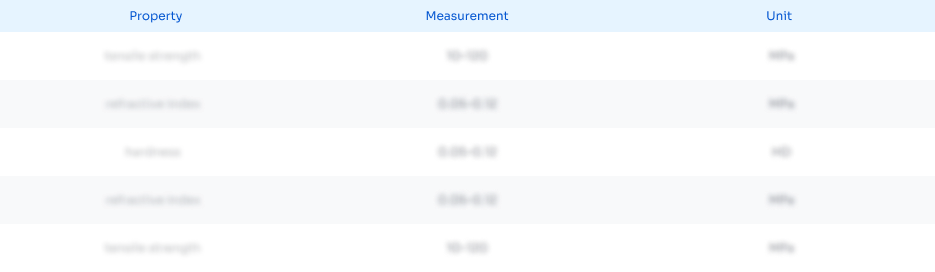
Abstract
Description
Claims
Application Information
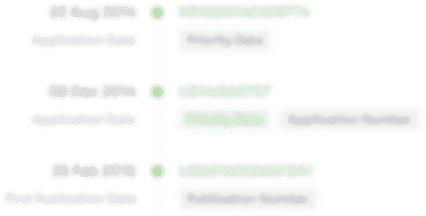
- R&D
- Intellectual Property
- Life Sciences
- Materials
- Tech Scout
- Unparalleled Data Quality
- Higher Quality Content
- 60% Fewer Hallucinations
Browse by: Latest US Patents, China's latest patents, Technical Efficacy Thesaurus, Application Domain, Technology Topic, Popular Technical Reports.
© 2025 PatSnap. All rights reserved.Legal|Privacy policy|Modern Slavery Act Transparency Statement|Sitemap|About US| Contact US: help@patsnap.com