Hyperspectral data dimensionality reduction method based on tensor distance patch alignment
A data dimensionality reduction and hyperspectral technology, applied in the field of hyperspectral remote sensing image processing, can solve the problems of data internal structure damage, high dimensionality, dimensionality disaster, etc.
- Summary
- Abstract
- Description
- Claims
- Application Information
AI Technical Summary
Problems solved by technology
Method used
Image
Examples
Embodiment 1
[0077] Example 1: The dimension reduction method is aimed at the tensor characteristics of hyperspectral data. First, the hyperspectral data is converted into a tensor form through the field formed by the central pixel and other similar pixels around it, that is, the "window field". , to maintain the spatial information of each pixel; second, introduce tensor distance to construct a high-quality tensor neighbor map containing discriminative information; third, obtain the globally optimal spectrum according to the patch calibration framework extended to tensor space- Spatial information; fourth, the solution of the tensor quantum space is obtained by using the alternating least squares algorithm; finally, the category of each sample is determined according to the tensor nearest neighbor method;
[0078] Specific steps are as follows:
[0079] Step 1, select the hyperspectral data to be analyzed, and convert the hyperspectral data into tensor form according to the window field;...
Embodiment 2
[0126] Embodiment 2: Through the AVIRIS92AV3C hyperspectral data experiment, the TDPA proposed by the present invention is compared with the existing MDA and MPCA tensor dimensionality reduction algorithms, and for the fairness of comparison, coordinate when seeking the neighbor distance of the high-quality tensor neighbor graph Parameter β=1. And according to the tensor nearest neighbor method to distinguish the category of each test sample, each experiment is done 20 times, and the average value is taken. Prove the superiority of TDPA.
[0127] combine figure 1 . The figure shows the key steps for dimensionality reduction processing of hyperspectral data using the tensor distance patch calibration method, which mainly includes seven steps: First: Calculate the tensor distance d between each training sample TD ; Second: Construct a high-quality tensor neighbor graph G according to the tensor distance; Third: Select χ according to the high-quality tensor neighbor graph i P...
PUM
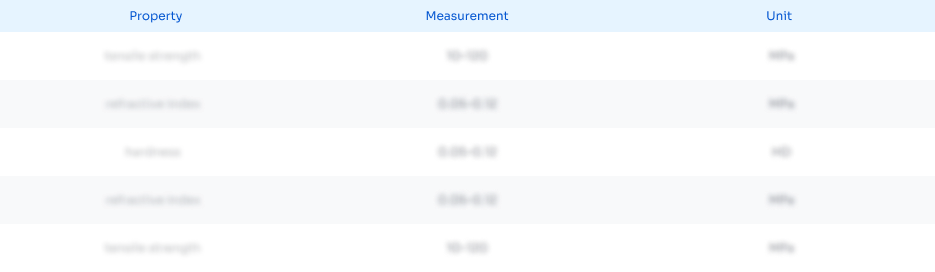
Abstract
Description
Claims
Application Information
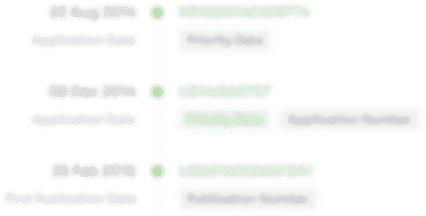
- R&D
- Intellectual Property
- Life Sciences
- Materials
- Tech Scout
- Unparalleled Data Quality
- Higher Quality Content
- 60% Fewer Hallucinations
Browse by: Latest US Patents, China's latest patents, Technical Efficacy Thesaurus, Application Domain, Technology Topic, Popular Technical Reports.
© 2025 PatSnap. All rights reserved.Legal|Privacy policy|Modern Slavery Act Transparency Statement|Sitemap|About US| Contact US: help@patsnap.com