Multi-channel spectrum clustering method based on local density estimation and neighbor relation spreading
A local density and density estimation technology, applied in the field of cluster analysis, can solve the problems that the affinity matrix cannot truly reflect the similarity relationship, the clustering results are inaccurate, and the similarity is reduced.
- Summary
- Abstract
- Description
- Claims
- Application Information
AI Technical Summary
Problems solved by technology
Method used
Image
Examples
Embodiment Construction
[0057] 1. Introduction to basic theory
[0058] 1. Spectrum theory
[0059] Assuming that each data sample is regarded as a vertex V in the graph, and the edges between vertices are assigned weights according to the similarity between samples, an undirected weighted graph G(V, E) based on sample similarity is constructed, then clustering The problem can be transformed into a graph partitioning problem.
[0060] The principle of graph partitioning is to maximize the weights in the subgraphs and minimize the weights between the subgraphs. Graph G is divided into V 1 and V 2 The cost function of the two subgraphs can be expressed as:
[0061] cut ( V 1 , V 2 ) = Σ u ∈ V 1 , v ...
PUM
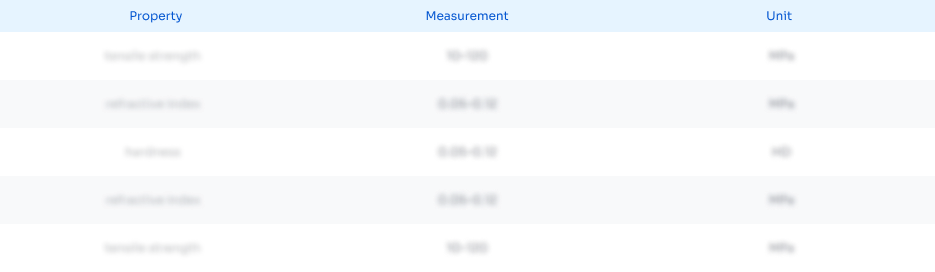
Abstract
Description
Claims
Application Information
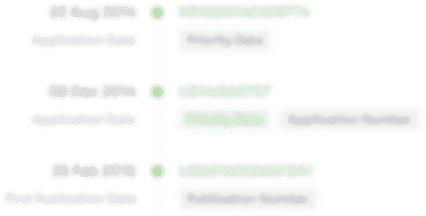
- R&D Engineer
- R&D Manager
- IP Professional
- Industry Leading Data Capabilities
- Powerful AI technology
- Patent DNA Extraction
Browse by: Latest US Patents, China's latest patents, Technical Efficacy Thesaurus, Application Domain, Technology Topic, Popular Technical Reports.
© 2024 PatSnap. All rights reserved.Legal|Privacy policy|Modern Slavery Act Transparency Statement|Sitemap|About US| Contact US: help@patsnap.com