Increment learning method on the basis of supporting vector geometrical significance
A support vector and incremental learning technology, applied in the direction of instruments, electrical digital data processing, character and pattern recognition, etc., can solve the problems of time-consuming classifiers and inability to meet real-time online, so as to meet online incremental learning tasks and avoid Repeat the iterative process to improve the effect of promotion ability
- Summary
- Abstract
- Description
- Claims
- Application Information
AI Technical Summary
Problems solved by technology
Method used
Image
Examples
Embodiment Construction
[0038] like Figure 1-Figure 3 Shown, the present invention is a kind of incremental learning method based on SVM geometric meaning.
[0039] Embodiments of the present invention adopt a computer with IntelCore-i3 central processing unit and 4G byte memory and use Matlab language to compile the work program based on the SVM incremental learning of convex hull vertex sample selection, and realize the method of the present invention.
[0040] The incremental learning classification method based on the SVM geometric meaning of the present invention mainly includes the following three steps: the design of the convex hull vertex sample selection method, the sample selection that can keep the convex hull information to the greatest extent, and the online update of the classifier. Proceed as follows:
[0041] (1) Propose an effective method for selecting convex hull vertex samples (called the CHVS method), that is, in a given sample set Select a given number of convex hull vertex ...
PUM
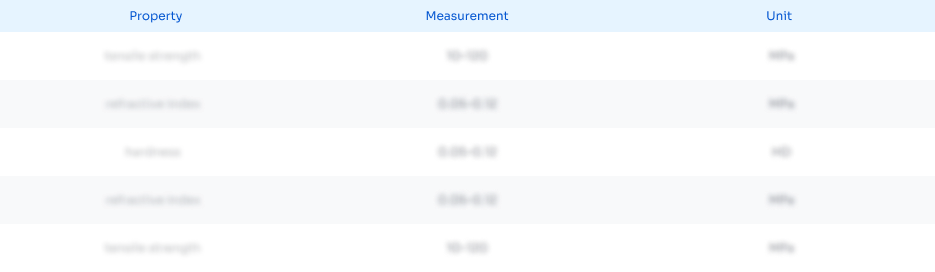
Abstract
Description
Claims
Application Information
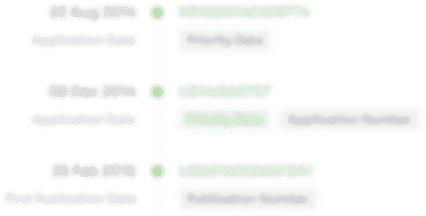
- R&D Engineer
- R&D Manager
- IP Professional
- Industry Leading Data Capabilities
- Powerful AI technology
- Patent DNA Extraction
Browse by: Latest US Patents, China's latest patents, Technical Efficacy Thesaurus, Application Domain, Technology Topic, Popular Technical Reports.
© 2024 PatSnap. All rights reserved.Legal|Privacy policy|Modern Slavery Act Transparency Statement|Sitemap|About US| Contact US: help@patsnap.com