Method for automatically annotating remote sensing images on basis of deep learning
A remote sensing image, automatic labeling technology, applied in special data processing applications, instruments, electrical digital data processing, etc.
- Summary
- Abstract
- Description
- Claims
- Application Information
AI Technical Summary
Problems solved by technology
Method used
Examples
Embodiment Construction
[0053] The present invention will be further described in detail below in conjunction with specific examples.
[0054] An automatic labeling method for remote sensing images based on deep learning, including:
[0055] (1) Extract the underlying feature vector of the remote sensing image to be labeled to construct the visual feature vector of the corresponding remote sensing image;
[0056] In this implementation, the underlying feature vectors include average spectral reflectance feature vectors, color layout description vectors, color structure description vectors, scalable color description vectors, homogeneous texture description vectors, edge histogram description vectors, GIST feature vectors, and SIFT feature-based Visual bag-of-words vectors.
[0057] The average spectral reflectance eigenvector can be obtained directly from the remote sensing image data, which is different from ordinary images, and the spectral information has been collected when the satellite shoots ...
PUM
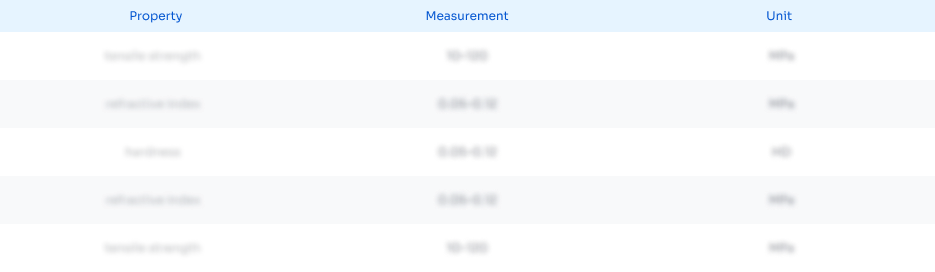
Abstract
Description
Claims
Application Information
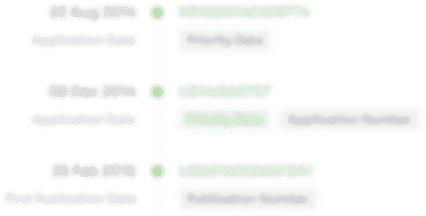
- R&D Engineer
- R&D Manager
- IP Professional
- Industry Leading Data Capabilities
- Powerful AI technology
- Patent DNA Extraction
Browse by: Latest US Patents, China's latest patents, Technical Efficacy Thesaurus, Application Domain, Technology Topic, Popular Technical Reports.
© 2024 PatSnap. All rights reserved.Legal|Privacy policy|Modern Slavery Act Transparency Statement|Sitemap|About US| Contact US: help@patsnap.com