Dictionary learning algorithm based on sparse model analysis
A dictionary learning and sparse model technology, applied in the field of signal processing, can solve problems such as estimating source signals in advance, achieve accurate target and background information, improve quality, and improve computing efficiency.
- Summary
- Abstract
- Description
- Claims
- Application Information
AI Technical Summary
Problems solved by technology
Method used
Image
Examples
Embodiment Construction
[0027] Preferred embodiments of the present invention are further explained in detail below.
[0028] The present invention directly uses the noise signal to construct the cost function, and uses the gradient descent method to solve the cost function, so as to ensure that the value solved by each iteration is optimal in a local range. The algorithm is used to update the dictionary adaptively to form a super-complete dictionary that can better represent the image structure. Then use the OBG algorithm to estimate the source signal for image denoising. The specific operation steps are as follows:
[0029] (1) Extract from the noisy image K indivual The size of the image sub-blocks, the sub-blocks are arranged in columns to obtain the training data matrix .
[0030] (2) Randomly generate an initial dictionary .
[0031] (3) Use the training data and the initial dictionary to construct an optimization function for solving sparse coefficients, as follows:
[0032] ...
PUM
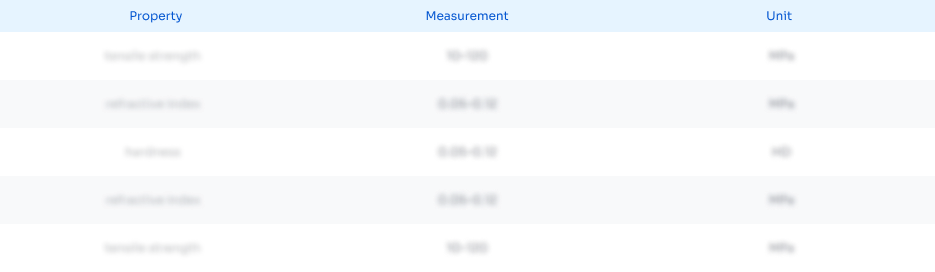
Abstract
Description
Claims
Application Information
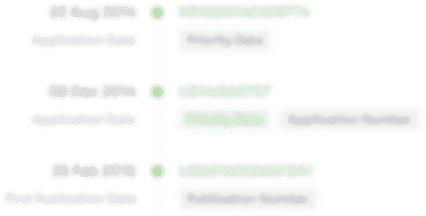
- R&D Engineer
- R&D Manager
- IP Professional
- Industry Leading Data Capabilities
- Powerful AI technology
- Patent DNA Extraction
Browse by: Latest US Patents, China's latest patents, Technical Efficacy Thesaurus, Application Domain, Technology Topic, Popular Technical Reports.
© 2024 PatSnap. All rights reserved.Legal|Privacy policy|Modern Slavery Act Transparency Statement|Sitemap|About US| Contact US: help@patsnap.com