Industrial fault diagnosis method based on improved KPCA (kernel principal component analysis) and hidden Markov model
A technology of fault diagnosis and modeling, applied in the direction of instruments, electrical testing/monitoring, control/regulation systems, etc., which can solve the problems of raw data redundancy, fault diagnosis errors, difficulty in nonlinear relationships, etc.
- Summary
- Abstract
- Description
- Claims
- Application Information
AI Technical Summary
Problems solved by technology
Method used
Image
Examples
Embodiment
[0101] As one of the most important basic industries in the national economy, iron and steel smelting is an important indicator to measure a country's economic level and comprehensive national strength. Blast furnace ironmaking is the most important link in the production process of the iron and steel industry, so it is of great significance to study the abnormal working condition diagnosis and safe operation methods of large blast furnaces.
[0102] The blast furnace is a huge airtight reaction vessel, and its internal smelting process is a typical "black box" operation through a series of complex physical, chemical and heat transfer reactions under high temperature and high pressure conditions. It is precisely because of the complexity inside the blast furnace that its monitoring process has the characteristics of nonlinear, non-Gaussian and multi-modal. Therefore, our proposed method is adaptable to blast furnace fault monitoring. The effectiveness of the method of the pre...
PUM
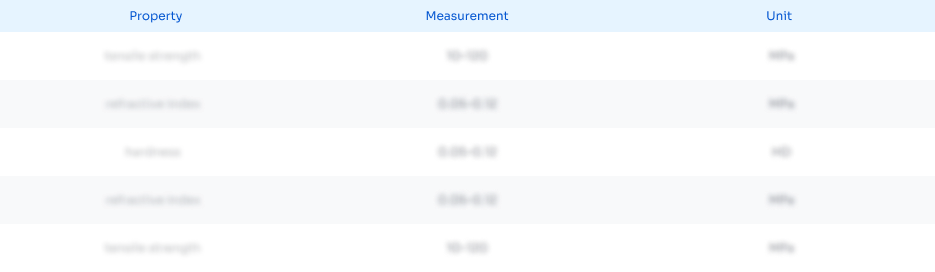
Abstract
Description
Claims
Application Information
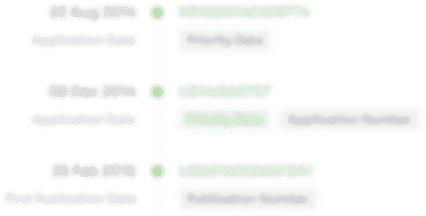
- R&D
- Intellectual Property
- Life Sciences
- Materials
- Tech Scout
- Unparalleled Data Quality
- Higher Quality Content
- 60% Fewer Hallucinations
Browse by: Latest US Patents, China's latest patents, Technical Efficacy Thesaurus, Application Domain, Technology Topic, Popular Technical Reports.
© 2025 PatSnap. All rights reserved.Legal|Privacy policy|Modern Slavery Act Transparency Statement|Sitemap|About US| Contact US: help@patsnap.com