Unsupervised hyperspectral data dimension reduction method based on block low-rank tensor model
A tensor model and data dimensionality reduction technology, applied in the field of image processing, can solve the problems of increasing application complexity, insignificant global spatial correlation, not considering hyperspectral images, etc., achieving economical and easy implementation, and improving the discrimination ability. Effect
- Summary
- Abstract
- Description
- Claims
- Application Information
AI Technical Summary
Problems solved by technology
Method used
Image
Examples
Embodiment Construction
[0038] The three-dimensional structure of the hyperspectral figure 1 As shown, the classic dimensionality reduction method needs to vectorize the three-dimensional structure and convert it into a two-dimensional matrix, thereby losing the spatial constraint information of the hyperspectral image, and resulting in a poor final dimensionality reduction effect, while the tensor representation used in the present invention Form, the hyperspectral three-dimensional structure is processed as a whole, the spatial constraint information of the hyperspectral image is well preserved, and the performance of the data dimensionality reduction method is improved.
[0039] The tensor, also known as multidimensional linear algebra, is a generalization of conventional linear algebra theory to high-dimensional spaces. tensor Represents a multidimensional array, where M is the order of this tensor, that is, the dimension of the multidimensional array, L i Then it represents the size of the te...
PUM
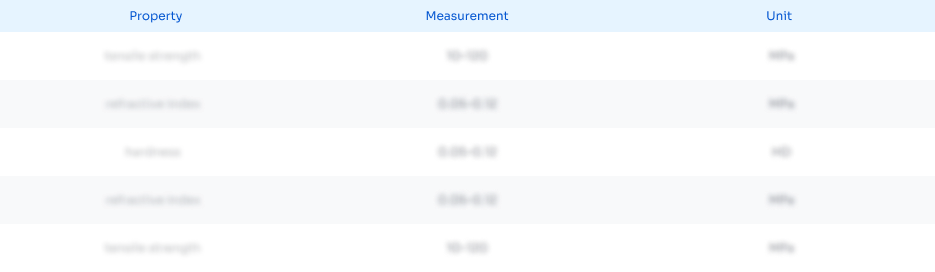
Abstract
Description
Claims
Application Information
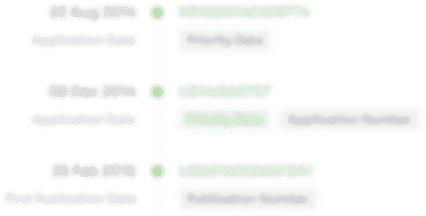
- Generate Ideas
- Intellectual Property
- Life Sciences
- Materials
- Tech Scout
- Unparalleled Data Quality
- Higher Quality Content
- 60% Fewer Hallucinations
Browse by: Latest US Patents, China's latest patents, Technical Efficacy Thesaurus, Application Domain, Technology Topic, Popular Technical Reports.
© 2025 PatSnap. All rights reserved.Legal|Privacy policy|Modern Slavery Act Transparency Statement|Sitemap|About US| Contact US: help@patsnap.com