Low-rank expression and learning dictionary-based hyperspectral image abnormity detection algorithm
A hyperspectral image, learning dictionary technology, applied in image enhancement, image analysis, image data processing and other directions, can solve the problems of abnormal target pollution of background covariance matrix and unsatisfactory PCA effect.
- Summary
- Abstract
- Description
- Claims
- Application Information
AI Technical Summary
Problems solved by technology
Method used
Image
Examples
Embodiment Construction
[0101] In the following, the specific implementation manners of the present invention will be described by taking simulated data and actual remote sensing image data as examples respectively.
[0102] The anomaly detection method based on low-rank representation and learning dictionary adopted in the present invention is denoted by LRRD.
[0103] 1. Simulation data experiment
[0104]The present invention adopts the method of embedding abnormal points in the hyperspectral image to construct the simulated experimental data. First, the influence of the learning dictionary on the LRR model is studied, and then the LRRD of the present invention is combined with the traditional GRX algorithm [2] and the literature [4]. The CRD algorithm based on co-expression is compared with two anomaly detection methods based on the low-rank matrix factorization algorithm RPCA and LRaSMD proposed in [8] and [10] to test the effectiveness of the proposed algorithm. The intuitive two-dimensional d...
PUM
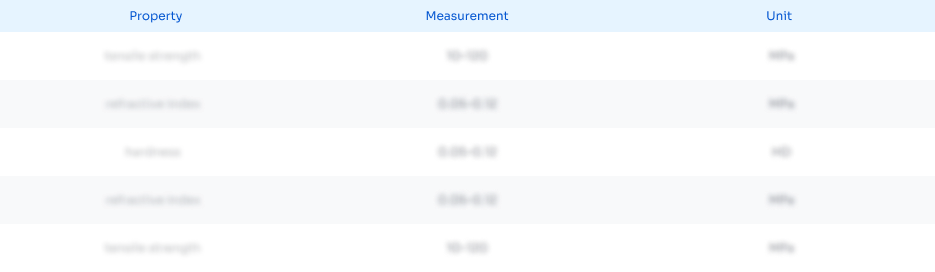
Abstract
Description
Claims
Application Information
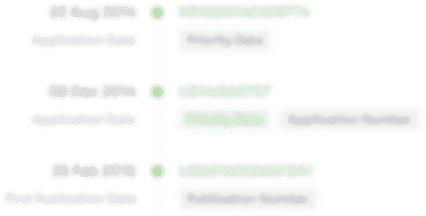
- R&D Engineer
- R&D Manager
- IP Professional
- Industry Leading Data Capabilities
- Powerful AI technology
- Patent DNA Extraction
Browse by: Latest US Patents, China's latest patents, Technical Efficacy Thesaurus, Application Domain, Technology Topic, Popular Technical Reports.
© 2024 PatSnap. All rights reserved.Legal|Privacy policy|Modern Slavery Act Transparency Statement|Sitemap|About US| Contact US: help@patsnap.com