Bearing fault diagnosis method and system based on improved LSSVM transfer learning
A technology of transfer learning and fault diagnosis, which is applied in the direction of mechanical bearing testing, mechanical component testing, machine/structural component testing, etc., can solve the problem that the distribution of marked data and target bearing fault data cannot be guaranteed, and reduce the traditional machine learning fault diagnosis. Model generalization ability, extra time and labor costs, etc.
- Summary
- Abstract
- Description
- Claims
- Application Information
AI Technical Summary
Problems solved by technology
Method used
Image
Examples
Embodiment Construction
[0143] like figure 1 and figure 2 As shown, the bearing fault diagnosis method based on transfer learning of the present invention mainly includes the following steps:
[0144] Step 1. Use recursive quantitative analysis (RQA) to extract nonlinear features from the target data and auxiliary data and combine them with traditional time-domain features to form feature vectors and form a training set.
[0145] In this step, the target data is the vibration data of the target bearing under the target working condition, and the source of auxiliary data is the vibration data of the target bearing under variable working conditions or the vibration data of adjacent bearings. The selection of auxiliary data mainly considers two factors: 1. Different bearing faults The commonality of deep causes (such as friction factors, contact surface factors, etc.); 2. The difference in working conditions (such as noise, load, etc.) is often manifested in the difference of a certain factor, but the...
PUM
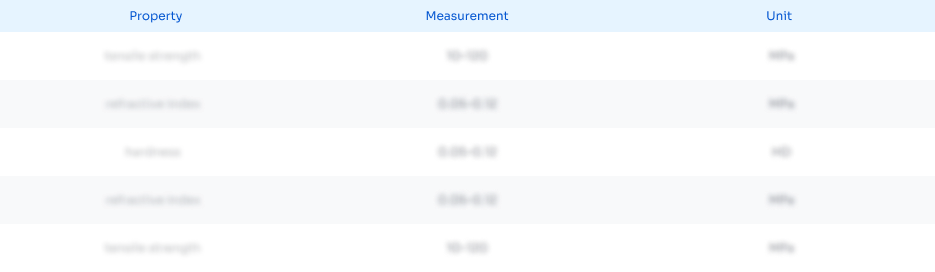
Abstract
Description
Claims
Application Information
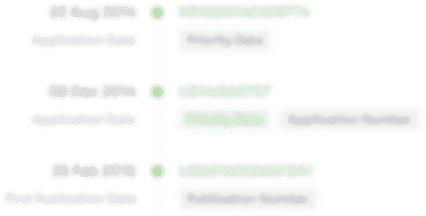
- R&D Engineer
- R&D Manager
- IP Professional
- Industry Leading Data Capabilities
- Powerful AI technology
- Patent DNA Extraction
Browse by: Latest US Patents, China's latest patents, Technical Efficacy Thesaurus, Application Domain, Technology Topic, Popular Technical Reports.
© 2024 PatSnap. All rights reserved.Legal|Privacy policy|Modern Slavery Act Transparency Statement|Sitemap|About US| Contact US: help@patsnap.com