Electroencephalogram feature classification method based on PSO-SVM (Particle Swarm Optimization-Support Vector Machine)
A PSO-SVM, EEG signal technology, applied in the direction of instruments, character and pattern recognition, computer components, etc., can solve the problems of lack of signal distribution characteristics, uncertainty of optimal kernel function selection, etc., to improve the classification recognition rate , the effect of high accuracy
- Summary
- Abstract
- Description
- Claims
- Application Information
AI Technical Summary
Problems solved by technology
Method used
Image
Examples
Embodiment Construction
[0034] The present invention will be further described below in conjunction with accompanying drawing.
[0035] The present invention comprises the following steps:
[0036] Step 1. Use the regularized CSP algorithm (R-CSP) to extract the features of the EEG signal to obtain the sample feature vector Y.
[0037] Step 2. Use the particle swarm optimization algorithm to iteratively optimize the kernel function parameters of the support vector machine.
[0038] Step 3. Use the optimal parameters after PSO optimization to train the SVM classifier, and use the trained classifier to classify and predict the samples.
[0039] Wherein step 1, the EEG signal feature extraction obtains the feature vector and the specific steps are as follows:
[0040] The regularized CSP algorithm is used to extract the features of the EEG signal, and the first and last q representative feature vectors are extracted from the two types of features, and the spatial filter is obtained as W. Let the motor...
PUM
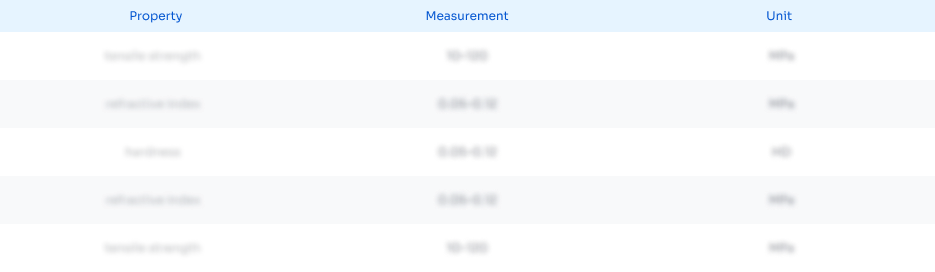
Abstract
Description
Claims
Application Information
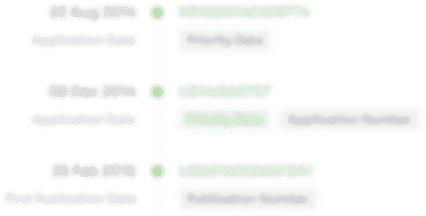
- R&D Engineer
- R&D Manager
- IP Professional
- Industry Leading Data Capabilities
- Powerful AI technology
- Patent DNA Extraction
Browse by: Latest US Patents, China's latest patents, Technical Efficacy Thesaurus, Application Domain, Technology Topic.
© 2024 PatSnap. All rights reserved.Legal|Privacy policy|Modern Slavery Act Transparency Statement|Sitemap