Transfer learning design method and system based on domain adaptation under multi-example multi-label framework
A multi-label learning and transfer learning technology, applied in computing, computer parts, character and pattern recognition, etc., can solve the problem of different distribution of sample sets, and achieve the solution of different distribution of sample sets, improve accuracy, and expand the scope of application. Effect
- Summary
- Abstract
- Description
- Claims
- Application Information
AI Technical Summary
Problems solved by technology
Method used
Image
Examples
Embodiment Construction
[0039] The invention will be described in further detail below in conjunction with the accompanying drawings.
[0040] Such as figure 1 As shown, the present invention provides a multi-instance multi-label transfer learning system based on domain adaptation, which includes a multi-instance sample single instantiation process module and a two-step domain adaptation process module.
[0041] The function of the multi-instance sample single instantiation module is to convert multi-instance multi-label samples into single-instance multi-label samples to facilitate domain adaptation.
[0042] The function of the two-step domain adaptation module is to weight and select the source domain samples so that they can be used for learning the target domain task.
[0043] Such as figure 2 As shown, the present invention provides a design method of multi-instance multi-label transfer learning based on domain adaptation, and the specific implementation steps of the method include the follo...
PUM
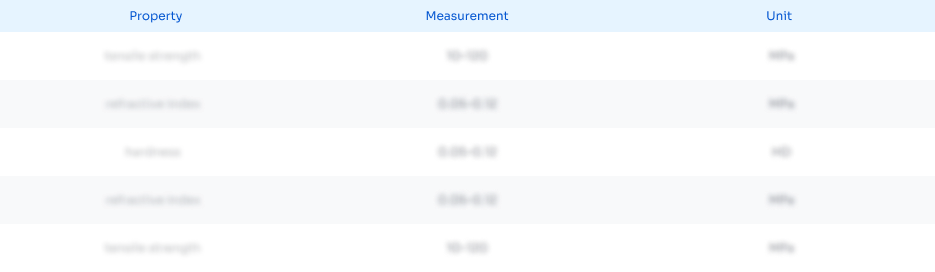
Abstract
Description
Claims
Application Information
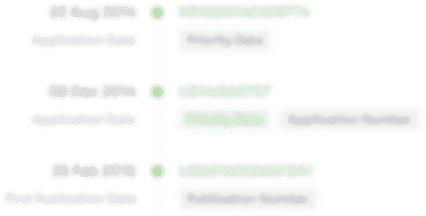
- R&D Engineer
- R&D Manager
- IP Professional
- Industry Leading Data Capabilities
- Powerful AI technology
- Patent DNA Extraction
Browse by: Latest US Patents, China's latest patents, Technical Efficacy Thesaurus, Application Domain, Technology Topic, Popular Technical Reports.
© 2024 PatSnap. All rights reserved.Legal|Privacy policy|Modern Slavery Act Transparency Statement|Sitemap|About US| Contact US: help@patsnap.com