Traffic scene classification method based on multi-scale convolution neural network
A convolutional neural network and traffic scene technology, applied in the field of road traffic scene classification in urban and suburban areas, to achieve increased accuracy, precise classification, and obvious training effects
- Summary
- Abstract
- Description
- Claims
- Application Information
AI Technical Summary
Problems solved by technology
Method used
Image
Examples
Embodiment Construction
[0033] The present invention will be further described below in conjunction with the accompanying drawings.
[0034] Such as figure 1 Shown, the specific embodiment of the present invention comprises the following steps:
[0035] A. Extract hidden features based on multi-scale convolutional neural network
[0036] A1. Based on the vehicle-mounted RGB-D camera, obtain the RGB-D image of the traffic scene in front of the vehicle, that is, color figure 1 and depth figure 2 , forming a four-channel Laplacian pyramid image 4 as the data input of the deep learning algorithm; at the same time, based on the image minimum spanning tree segmentation, using the classic region fusion method, taking the RGB-D image in the traffic scene as the input, the structure has a hierarchical structure The original split tree of 3. Among them, each node in the original segmentation tree 3 corresponds to an original classification image area, and the root node C 10 Represents the entire origin...
PUM
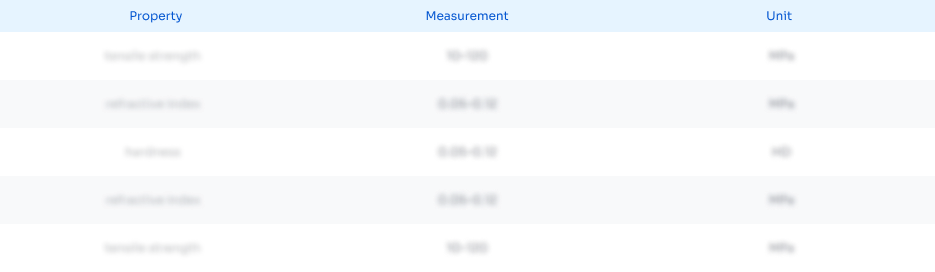
Abstract
Description
Claims
Application Information
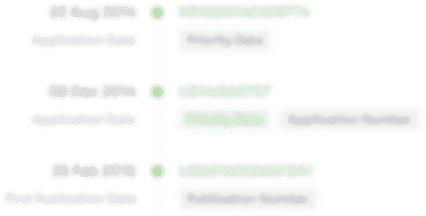
- Generate Ideas
- Intellectual Property
- Life Sciences
- Materials
- Tech Scout
- Unparalleled Data Quality
- Higher Quality Content
- 60% Fewer Hallucinations
Browse by: Latest US Patents, China's latest patents, Technical Efficacy Thesaurus, Application Domain, Technology Topic, Popular Technical Reports.
© 2025 PatSnap. All rights reserved.Legal|Privacy policy|Modern Slavery Act Transparency Statement|Sitemap|About US| Contact US: help@patsnap.com