Analog circuit fault diagnosis method based on depth learning and complex characteristics
A technology for simulating circuit faults and diagnostic methods, which is applied in the direction of analog circuit testing, electronic circuit testing, and electrical measurement. It can solve problems such as complex objective functions, error diffusion, and training failures, and achieve the effect of enriching sample information and improving accuracy.
- Summary
- Abstract
- Description
- Claims
- Application Information
AI Technical Summary
Problems solved by technology
Method used
Image
Examples
Embodiment
[0023] In order to better illustrate the technical solution of the present invention, firstly, the deep learning model on which the present invention is based is briefly described.
[0024] figure 1 is the structural diagram of the autoencoder network model. Such as figure 1 As shown, the self-encoder neural network tries to learn a h W,b (x) ≈ a function of x. In other words, it tries to approximate an identity function such that the output close to the input x. When the number of neurons in the hidden layer L2 is specified to be less than the number of neurons in the input layer L1, this means that the self-encoder neural network is forced to learn a compressed representation of the input data. If there is some specific structure implied in the input data, such as certain input features are related to each other, then this algorithm can discover these correlations in the input data. If the number of neurons in the hidden layer is greater than the number of neurons in ...
PUM
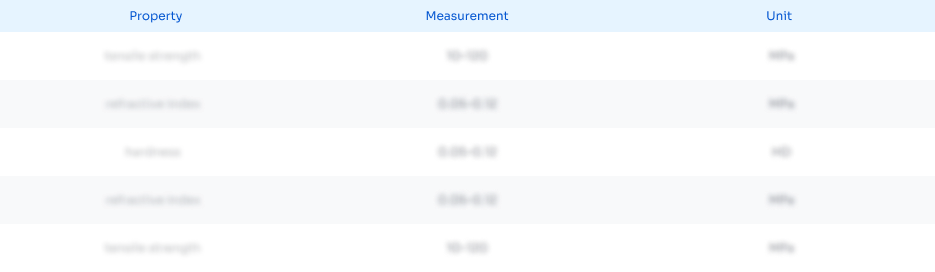
Abstract
Description
Claims
Application Information
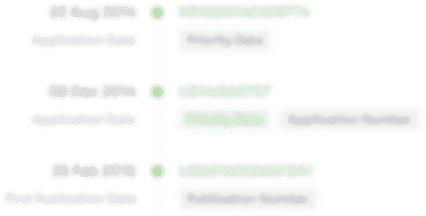
- R&D Engineer
- R&D Manager
- IP Professional
- Industry Leading Data Capabilities
- Powerful AI technology
- Patent DNA Extraction
Browse by: Latest US Patents, China's latest patents, Technical Efficacy Thesaurus, Application Domain, Technology Topic, Popular Technical Reports.
© 2024 PatSnap. All rights reserved.Legal|Privacy policy|Modern Slavery Act Transparency Statement|Sitemap|About US| Contact US: help@patsnap.com