A Method of Object Classification and Pose Detection Based on Deep Convolutional Neural Network
A deep convolution and target classification technology, applied in biological neural network model, neural architecture, image analysis and other directions, can solve the problems of limited detection ability, decreased signal-to-noise ratio, inability to characterize posture, etc., to improve the accuracy and complete the characterization. , to avoid the effect of a large number of calculations
- Summary
- Abstract
- Description
- Claims
- Application Information
AI Technical Summary
Problems solved by technology
Method used
Image
Examples
Embodiment Construction
[0032] In order to express the purpose, technical solutions and advantages of the present invention more clearly, the following in conjunction with specific embodiments, and with reference to the accompanying drawings, provides a further detailed description of the present invention, but the scope of protection of the present invention is not limited to the following embodiments.
[0033] The framework for detecting the target in this embodiment is as follows figure 1 As shown in Fig. 1, after the deep convolutional features of the image are obtained through the convolutional neural network, the candidate window with the attitude angle is mapped to the feature layer to obtain the directional regional feature vector, and then the final feature vector is obtained by classifying and predicting the feature vector. Test results.
[0034] The data sets used in this example are partially derived from the public image databases PASCAL VOC 2007 and PASCAL VOC2012. In addition, we have ...
PUM
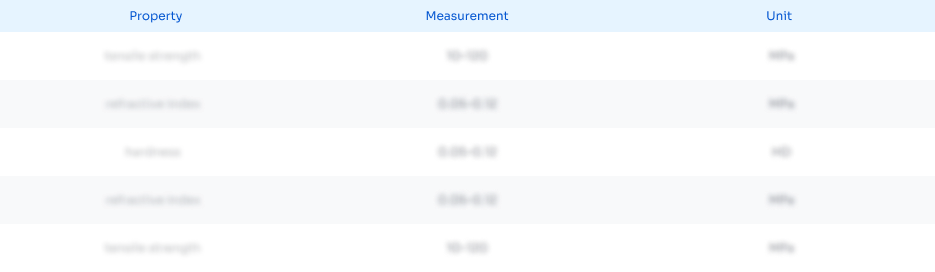
Abstract
Description
Claims
Application Information
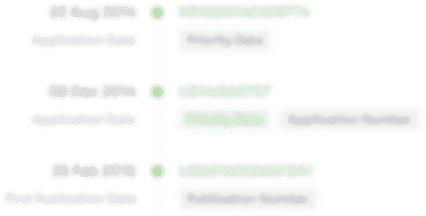
- R&D
- Intellectual Property
- Life Sciences
- Materials
- Tech Scout
- Unparalleled Data Quality
- Higher Quality Content
- 60% Fewer Hallucinations
Browse by: Latest US Patents, China's latest patents, Technical Efficacy Thesaurus, Application Domain, Technology Topic, Popular Technical Reports.
© 2025 PatSnap. All rights reserved.Legal|Privacy policy|Modern Slavery Act Transparency Statement|Sitemap|About US| Contact US: help@patsnap.com