Pedestrian image feature classification method and system
A technology of image features and classification methods, applied in the field of image processing, can solve problems such as poor robustness, low recognition rate, and inability to guarantee robustness, and achieve the effect of high practicability and guaranteed robustness
- Summary
- Abstract
- Description
- Claims
- Application Information
AI Technical Summary
Problems solved by technology
Method used
Image
Examples
Embodiment Construction
[0053] The principles and features of the present invention are described below in conjunction with the accompanying drawings, and the examples given are only used to explain the present invention, and are not intended to limit the scope of the present invention.
[0054] figure 1 A method flowchart of a pedestrian image feature classification method provided by an embodiment of the present invention;
[0055] Such as figure 1 As shown, a pedestrian image feature classification method, including:
[0056] Step S1: Perform image mirroring processing on the pedestrian image samples in the sample data set to expand the sample data set; the sample data sets are CUHK01 library and VIPER library;
[0057] Step S2: Group the pedestrian image samples in the expanded sample data set, divide the pedestrian image samples and mirror images belonging to the same pedestrian into the same sample group, and obtain multiple pedestrian sample groups;
[0058] Step S3: Select a pedestrian ima...
PUM
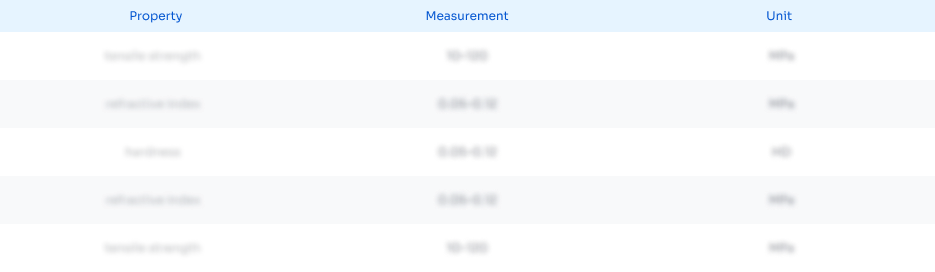
Abstract
Description
Claims
Application Information
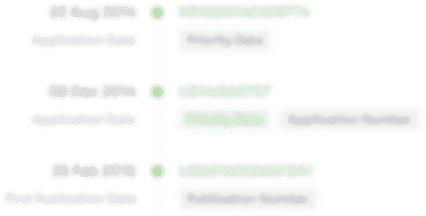
- R&D Engineer
- R&D Manager
- IP Professional
- Industry Leading Data Capabilities
- Powerful AI technology
- Patent DNA Extraction
Browse by: Latest US Patents, China's latest patents, Technical Efficacy Thesaurus, Application Domain, Technology Topic, Popular Technical Reports.
© 2024 PatSnap. All rights reserved.Legal|Privacy policy|Modern Slavery Act Transparency Statement|Sitemap|About US| Contact US: help@patsnap.com