Clustering analysis-based intelligent fault diagnosis method for antifriction bearing of mechanical system
A mechanical system and rolling bearing technology, applied in the field of equipment system fault monitoring and diagnosis, can solve problems such as low identification and calculation efficiency, signal classification and extraction of fault information features, and failure to complete fault diagnosis.
- Summary
- Abstract
- Description
- Claims
- Application Information
AI Technical Summary
Problems solved by technology
Method used
Image
Examples
Embodiment Construction
[0136] The content of the present invention will be further described in detail below in conjunction with the accompanying drawings and specific embodiments.
[0137] This embodiment adopts the intelligent fault diagnosis method of mechanical system rolling bearings based on cluster analysis of the present invention, and according to the vibration signal of a single bearing, the vibration signals of outer ring faults, inner ring faults, rolling element faults, cage faults and normal bearings Analyze and finally give the diagnosis result.
[0138] An example of the implementation of the intelligent fault diagnosis method for rolling bearings in mechanical systems based on cluster analysis figure 1 As shown, it includes two parts. The first part is to train the diagnostic model, and the second part is to use the trained diagnostic model to diagnose the rolling bearing in the mechanical system.
[0139] The main steps of the first part of training the diagnostic model are as fol...
PUM
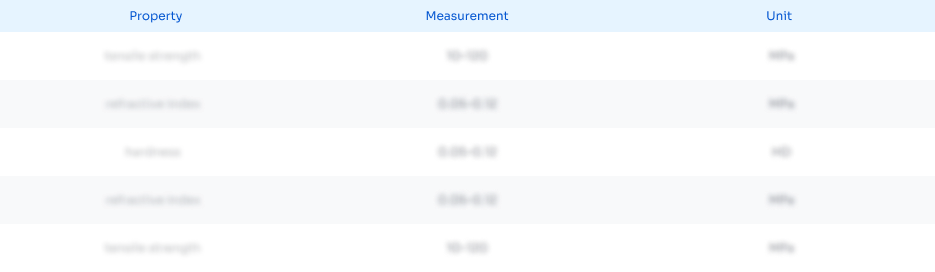
Abstract
Description
Claims
Application Information
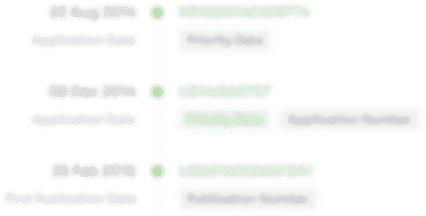
- R&D Engineer
- R&D Manager
- IP Professional
- Industry Leading Data Capabilities
- Powerful AI technology
- Patent DNA Extraction
Browse by: Latest US Patents, China's latest patents, Technical Efficacy Thesaurus, Application Domain, Technology Topic, Popular Technical Reports.
© 2024 PatSnap. All rights reserved.Legal|Privacy policy|Modern Slavery Act Transparency Statement|Sitemap|About US| Contact US: help@patsnap.com