Neural network method for predicting river flow rate based on multi-section water levels
A neural network and BP neural network technology, applied in the field of neural network for predicting river flow based on multi-section water level, can solve the problem of breaking the one-to-one correspondence between water level and flow, river sections not meeting the conditions of constant flow, and single-value curve fitting Poor accuracy and other problems, to achieve the effect of solving the problem of water level-flow curve, easy to promote, and high accuracy
- Summary
- Abstract
- Description
- Claims
- Application Information
AI Technical Summary
Problems solved by technology
Method used
Image
Examples
Embodiment
[0034] The present invention uses different water level stations to measure the water levels on different sections of the river, and the multi-section and multi-water level stations should be essentially the same. What the present invention obtains at last is the water level-flow relationship, so hydraulic gradient is not involved in the relationship, but due to the change of hydraulic gradient in the year, multi-valued rope loop type curves (such as figure 1 The water level-discharge relationship of the Cuntan water level station in 2013 is completely different from the general power function relationship). At this time, we still finally want to obtain the water level-flow relationship. The present invention uses more than three water level data to reflect the change of hydraulic gradient, that is, one water level station is selected at the upstream and downstream of the Cuntan hydrological station, which are Xuantan Temple and Xuantan Temple respectively. Tongluoxia Water Le...
PUM
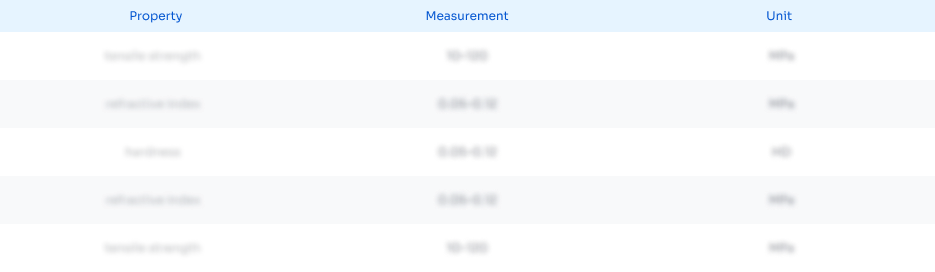
Abstract
Description
Claims
Application Information
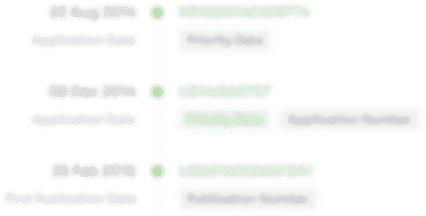
- R&D Engineer
- R&D Manager
- IP Professional
- Industry Leading Data Capabilities
- Powerful AI technology
- Patent DNA Extraction
Browse by: Latest US Patents, China's latest patents, Technical Efficacy Thesaurus, Application Domain, Technology Topic, Popular Technical Reports.
© 2024 PatSnap. All rights reserved.Legal|Privacy policy|Modern Slavery Act Transparency Statement|Sitemap|About US| Contact US: help@patsnap.com