Method for re-identifying persons on basis of deep learning encoding models
A coding model and deep learning technology, applied in the field of person re-identification based on the deep learning coding model, can solve problems such as poor effect, high computational complexity of the classifier, and poor quality
- Summary
- Abstract
- Description
- Claims
- Application Information
AI Technical Summary
Problems solved by technology
Method used
Image
Examples
Embodiment 1
[0056] Embodiment 1: The person re-identification method based on the deep learning coding model in this embodiment has serious quantization errors for vector quantization coding, and sparse coding is only a shallow learning model, which easily leads to the lack of selectivity of visual dictionaries for image features. First, a deep learning network—unsupervised Restricted Boltzmann Machine (RBM) is used to replace the traditional K-Means clustering and sparse coding methods to encode and learn the SIFT feature library to generate a visual dictionary; secondly, according to the learned The dictionary, get the sparse vector corresponding to each SIFT feature, and fuse it to get the deep learning representation vector of the image, and use it to train the SVM classifier; then, use the category label information of the training data to supervise the RBM network learning fine-tuning, and use the SVM classifier to complete the classification and recognition of pedestrians.
[0057]...
Embodiment 2
[0058] Embodiment two: see figure 2 , image 3 The person re-identification method based on the deep learning coding model of this embodiment adopts the following steps to generate a visual dictionary with both sparsity and selectivity:
[0059] First, extract the SIFT features of the training image library; extract the SIFT features; secondly, combine the spatial information of the SIFT features, use the adjacent SIFT features as the input of the RBM, train the RBM through the CD fast algorithm, and obtain the hidden layer features; then the adjacent hidden layer The features are used as the input of the next layer of RBM to get the output dictionary. Among them, ω 1 and ω 2 is the connection weight of RBM. RBM has an obvious layer and a hidden layer, but in RBM, there is no connection between neurons in the same layer, so the learning process is simpler.
[0060] During the training process of the network, the hidden layer and the visible layer of RBM are related by the...
Embodiment 3
[0076] Embodiment three: see Figure 4 , in order to express the image content more accurately in this embodiment, a regular term h(z) is added to the RBM objective optimization function, and the objective function Adjust as follows:
[0077]
[0078] Among them, λ is the weighting coefficient of the regular term. Deep learning coding can make the learned visual dictionary more selective, and make the image expression vector have better sparsity.
[0079] The sparsity and selectivity can be quantitatively analyzed by using the mean value of the visual dictionary’s response to each dimension feature, namely:
[0080]
[0081] in, is the expected value of the average activation probability of each word for K features, word z j for feature x k The expected value of the response probability can be denoted as p jk ∈(0,1), then the expected response value of the entire dictionary to K input features can be written as a matrix Each row element p in the matrix j· repr...
PUM
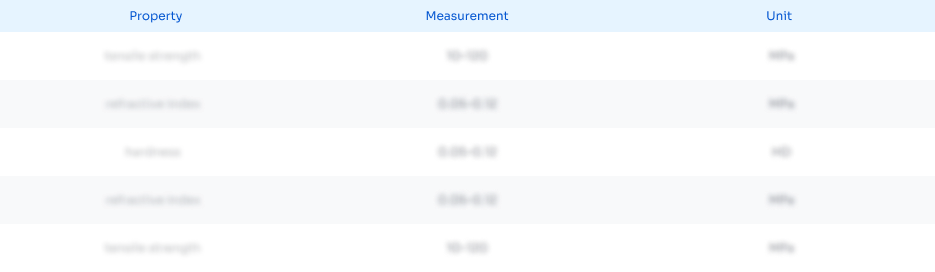
Abstract
Description
Claims
Application Information
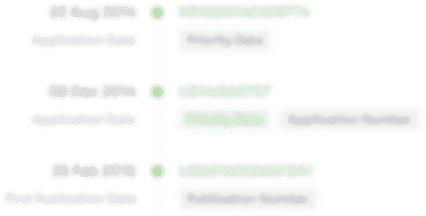
- R&D Engineer
- R&D Manager
- IP Professional
- Industry Leading Data Capabilities
- Powerful AI technology
- Patent DNA Extraction
Browse by: Latest US Patents, China's latest patents, Technical Efficacy Thesaurus, Application Domain, Technology Topic, Popular Technical Reports.
© 2024 PatSnap. All rights reserved.Legal|Privacy policy|Modern Slavery Act Transparency Statement|Sitemap|About US| Contact US: help@patsnap.com