Deep-convolution-neural-network-based super-resolution reconstruction method of single image
A technology of super-resolution reconstruction and deep convolution, which is applied in the field of digital image processing, can solve problems such as slow calculation speed and complex structure, and achieve the effect of less image preprocessing, good restoration quality and fast speed
- Summary
- Abstract
- Description
- Claims
- Application Information
AI Technical Summary
Problems solved by technology
Method used
Image
Examples
Embodiment
[0031] A single image super-resolution reconstruction method based on a deep convolutional neural network, comprising the following steps:
[0032] (1) if figure 1 As shown, the image preprocessing step includes two processes: converting the input image from the RGB color space to the YCbCr color space, and extracting the Y channel in the YCbCr color space, that is, the brightness channel as the preprocessed image;
[0033] (2) if figure 2 As shown, the preprocessed image obtained in step (1) is down-sampled to form a low-resolution image, and then interpolated in two channels:
[0034] (2.1) Channel 1 performs bicubic interpolation, then densely extracts small blocks, and uses these small blocks as channel 1 training data;
[0035] (2.2) Passage 2 carries out the nearest neighbor interpolation, and the bicubic interpolation result of the passage 1 of the result and described step (2.1) is multiplied as a mask, then densely extracts small blocks, these small blocks are used...
PUM
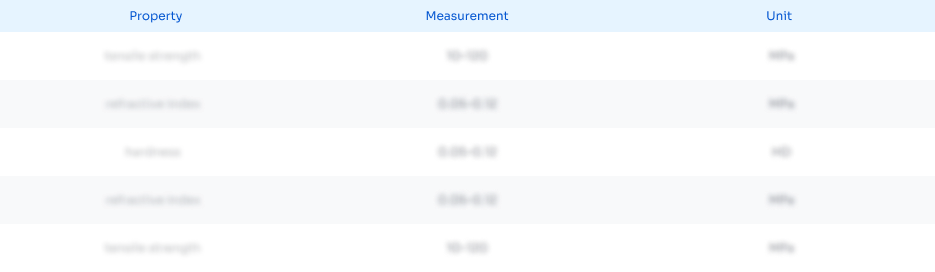
Abstract
Description
Claims
Application Information
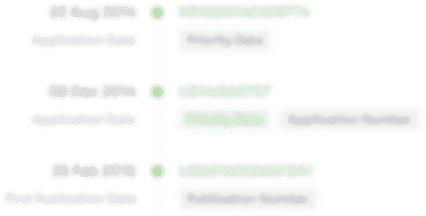
- R&D
- Intellectual Property
- Life Sciences
- Materials
- Tech Scout
- Unparalleled Data Quality
- Higher Quality Content
- 60% Fewer Hallucinations
Browse by: Latest US Patents, China's latest patents, Technical Efficacy Thesaurus, Application Domain, Technology Topic, Popular Technical Reports.
© 2025 PatSnap. All rights reserved.Legal|Privacy policy|Modern Slavery Act Transparency Statement|Sitemap|About US| Contact US: help@patsnap.com