Deep learning network model for travel time prediction and method for establishing same
A travel time and prediction method technology, applied in traffic flow detection, traffic control systems for road vehicles, instruments, etc., can solve problems such as poor robustness and accuracy, strong uncertainty, and large influence of random interference factors.
- Summary
- Abstract
- Description
- Claims
- Application Information
AI Technical Summary
Problems solved by technology
Method used
Image
Examples
Embodiment Construction
[0054] The present invention is further described below in conjunction with embodiment.
[0055] The present invention provides a travel time prediction model based on floating car data considering the time-space relationship of traffic status and its establishment method. The process is as follows figure 1 shown. Taking the Second Ring Expressway in Beijing as an example below, the process of establishing the travel time prediction model of the present invention will be described in detail. The total length of the Second Ring Expressway in Beijing is 32.7km. A total of 45 days of floating car historical data were extracted from 6:00 am to 10:00 pm on the 14th, and the method proposed by the present invention was used to establish, train and verify the travel time prediction model. The various steps of the modeling are described in detail below.
[0056] Step 1) Floating car data processing.
[0057] The road network including the Second Ring Road in Beijing is divided into ...
PUM
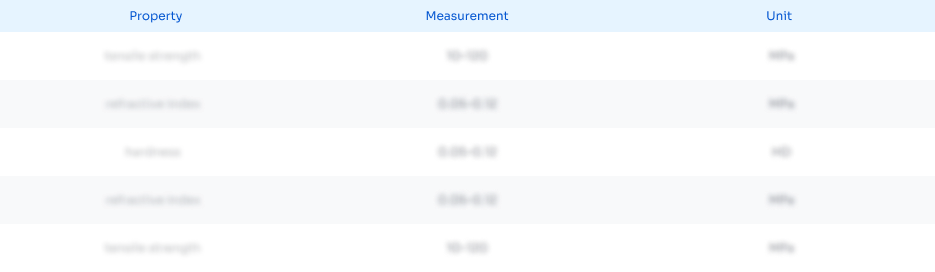
Abstract
Description
Claims
Application Information
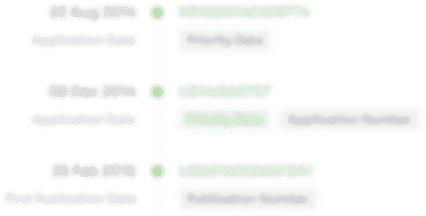
- Generate Ideas
- Intellectual Property
- Life Sciences
- Materials
- Tech Scout
- Unparalleled Data Quality
- Higher Quality Content
- 60% Fewer Hallucinations
Browse by: Latest US Patents, China's latest patents, Technical Efficacy Thesaurus, Application Domain, Technology Topic, Popular Technical Reports.
© 2025 PatSnap. All rights reserved.Legal|Privacy policy|Modern Slavery Act Transparency Statement|Sitemap|About US| Contact US: help@patsnap.com