Deep-convolution-neural-network-based CT pulmonary nodule detection method
A neural network and deep convolution technology, applied in the field of CT pulmonary nodule detection based on deep convolutional neural network, can solve the problems of shallow network structure and failure to make full use of deep learning, etc., achieve strong adaptability and improve prediction ability , high efficiency effect
- Summary
- Abstract
- Description
- Claims
- Application Information
AI Technical Summary
Problems solved by technology
Method used
Image
Examples
Embodiment Construction
[0042] The specific implementation manners of the present invention will be further described in detail below in conjunction with the accompanying drawings and embodiments. The following examples are used to illustrate the present invention, but are not intended to limit the scope of the invention:
[0043] The data used in the specific experiments of the present invention come from the LIDC / IDRI database, including CT images of 888 patients, including 1086 nodules in total. The data was obtained by four experienced chest radiologists who performed two image annotations on the patient's CT images. The first time was blind labeling, and the second time was to refer to other doctors to correct the results. However, these 888 CT images are collected by different instruments, and the pixel intervals of CT images are different, and the range of variation is large. 0.46-0.97mm. At the same time, the HU range and contrast of these CT images are also different.
[0044] Such as fi...
PUM
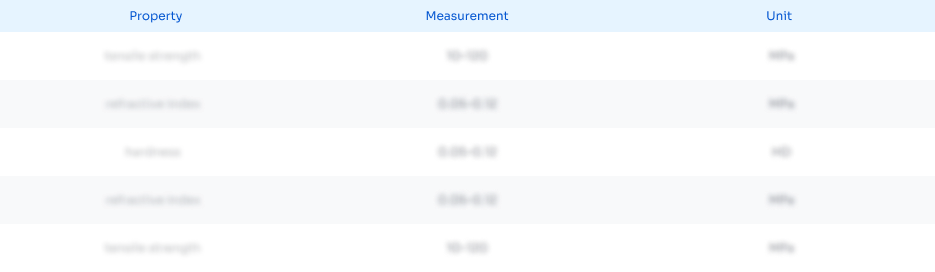
Abstract
Description
Claims
Application Information
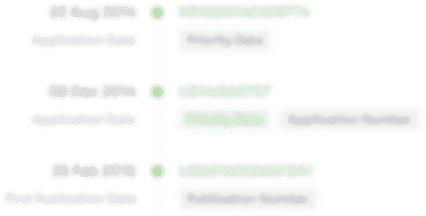
- Generate Ideas
- Intellectual Property
- Life Sciences
- Materials
- Tech Scout
- Unparalleled Data Quality
- Higher Quality Content
- 60% Fewer Hallucinations
Browse by: Latest US Patents, China's latest patents, Technical Efficacy Thesaurus, Application Domain, Technology Topic, Popular Technical Reports.
© 2025 PatSnap. All rights reserved.Legal|Privacy policy|Modern Slavery Act Transparency Statement|Sitemap|About US| Contact US: help@patsnap.com