Turbulent image de-noising method
An image and turbulence technology, applied in the field of image processing, can solve problems such as large computational complexity, slow convergence, and target image distortion
- Summary
- Abstract
- Description
- Claims
- Application Information
AI Technical Summary
Problems solved by technology
Method used
Image
Examples
Embodiment Construction
[0060] In order to make the object, technical solution and advantages of the present invention clearer, the implementation manner of the present invention will be further described in detail below in conjunction with the accompanying drawings.
[0061] The present invention provides a turbulent image denoising method, see figure 1 , including the following steps:
[0062] S100: performing single-layer two-dimensional discrete wavelet transform on the noise-containing turbulence image to obtain reconstructed low-frequency and high-frequency coefficients;
[0063] Specifically, performing single-layer two-dimensional discrete wavelet transform on the noisy turbulent image to obtain reconstructed low-frequency and high-frequency coefficients is specifically:
[0064] The Mallat algorithm is used to perform single-layer 2-D discrete wavelet transform on the turbulence degradation image, decompose it into 4 subbands, extract the decomposed low-frequency and high-frequency coeffici...
PUM
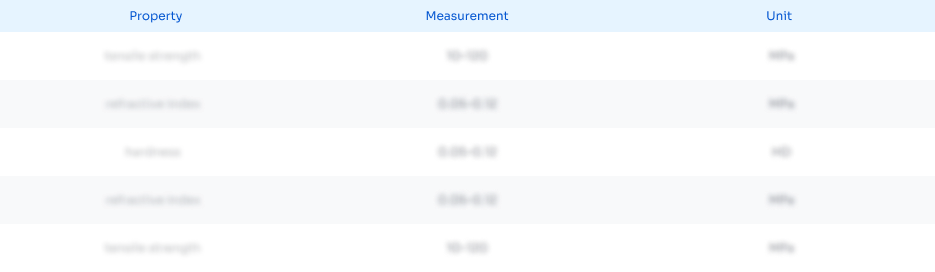
Abstract
Description
Claims
Application Information
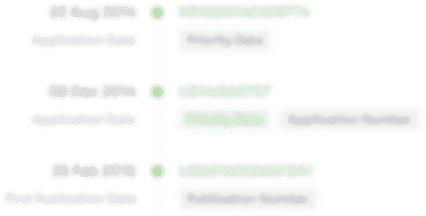
- R&D Engineer
- R&D Manager
- IP Professional
- Industry Leading Data Capabilities
- Powerful AI technology
- Patent DNA Extraction
Browse by: Latest US Patents, China's latest patents, Technical Efficacy Thesaurus, Application Domain, Technology Topic, Popular Technical Reports.
© 2024 PatSnap. All rights reserved.Legal|Privacy policy|Modern Slavery Act Transparency Statement|Sitemap|About US| Contact US: help@patsnap.com