Polarized SAR land feature classification method based on full convolution neural network
A convolutional neural network and ground object classification technology, applied in the direction of neural learning methods, biological neural network models, instruments, etc., can solve the problems of reducing the processing time of polarization SAR image classification, classification duration, and many classification duration parameters, etc., to achieve Improve test accuracy and running time, improve running time, and avoid edge effects
- Summary
- Abstract
- Description
- Claims
- Application Information
AI Technical Summary
Problems solved by technology
Method used
Image
Examples
Embodiment Construction
[0059] Below in conjunction with accompanying drawing and embodiment the realization step of the present invention and experimental effect are described in further detail:
[0060] refer to figure 1 , the specific implementation steps of the present invention are as follows:
[0061] Step 1: Carry out Pauli decomposition on the polarized scattering matrix S to obtain the odd-order scattering coefficient, even-order scattering coefficient and volume scattering coefficient, and use the odd-order scattering coefficient, even-order scattering coefficient and volume scattering coefficient as the three-dimensional The image feature matrix F specifically includes the following steps:
[0062] (1a) Define the Pauli basis {S 1 ,S 2 ,S 3} is the formula , and the formula is as follows:
[0063]
[0064] where S 1 Indicates odd scattering, S 2 Indicates even scattering, S 3 Indicates volume scattering;
[0065] (1b) Equation is obtained by Pauli decomposition definition, an...
PUM
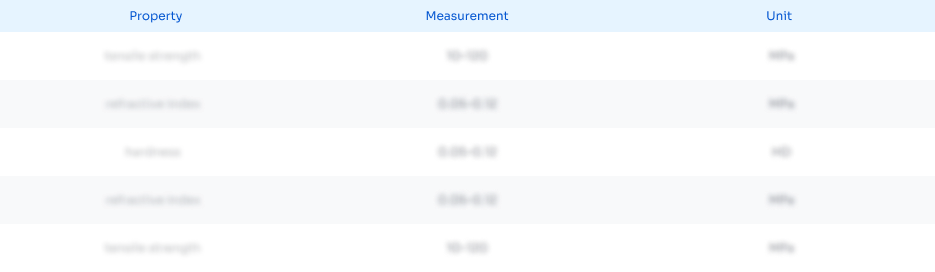
Abstract
Description
Claims
Application Information
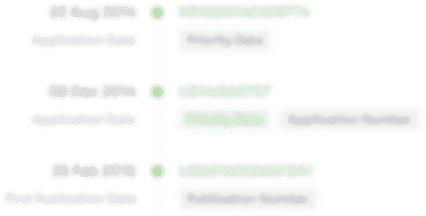
- R&D Engineer
- R&D Manager
- IP Professional
- Industry Leading Data Capabilities
- Powerful AI technology
- Patent DNA Extraction
Browse by: Latest US Patents, China's latest patents, Technical Efficacy Thesaurus, Application Domain, Technology Topic, Popular Technical Reports.
© 2024 PatSnap. All rights reserved.Legal|Privacy policy|Modern Slavery Act Transparency Statement|Sitemap|About US| Contact US: help@patsnap.com