Multi-aspect deep learning expression-based image emotion classification method
A technology of emotion classification and deep learning, applied to instruments, character and pattern recognition, computer components, etc., to achieve the effects of reducing a lot of time, improving accuracy, and improving robustness
- Summary
- Abstract
- Description
- Claims
- Application Information
AI Technical Summary
Problems solved by technology
Method used
Image
Examples
Embodiment
[0051] Such as figure 1 As shown, the image emotion classification method based on multi-faceted deep learning expression of this implementation includes the following steps:
[0052] (1) Image sentiment classification model design: including a parallel convolutional neural network model (such as image 3 shown) and a support vector machine (SVM) classifier for decision fusion network features.
[0053] (2) The structural design of the parallel convolutional neural network model, the specific network parameter settings and methods are as follows:
[0054] Such as figure 2 As shown, the model of the present invention contains 5 mutually independent networks, and each network structure is the same, borrowing ResNet-50-layer [K.He, X.Zhang, S.Ren, et al, Deep Residual Learning for ImageRecognition, Structure and parameters of IEEE Conference on Computer Vision and Pattern Recognition (CVPR), pp:770-778, 2016.]. Each network contains 5 layers of convolutional layers, 1 fully ...
PUM
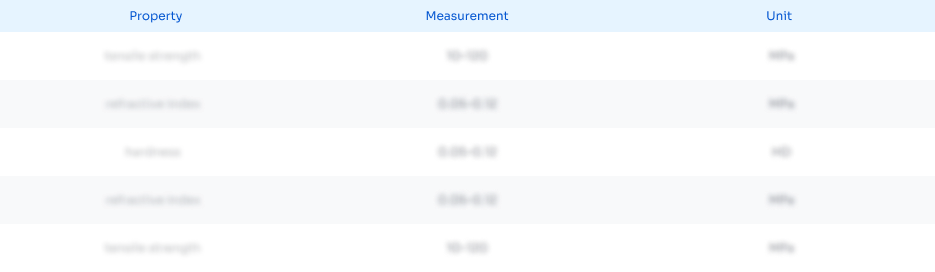
Abstract
Description
Claims
Application Information
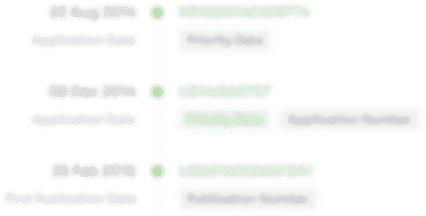
- R&D Engineer
- R&D Manager
- IP Professional
- Industry Leading Data Capabilities
- Powerful AI technology
- Patent DNA Extraction
Browse by: Latest US Patents, China's latest patents, Technical Efficacy Thesaurus, Application Domain, Technology Topic.
© 2024 PatSnap. All rights reserved.Legal|Privacy policy|Modern Slavery Act Transparency Statement|Sitemap