Hypersonic aerocraft neural network composite learning control method based on robustness design
A hypersonic, learning control technology, applied in the direction of adaptive control, general control system, control/regulation system, etc., can solve problems such as poor practicability
- Summary
- Abstract
- Description
- Claims
- Application Information
AI Technical Summary
Problems solved by technology
Method used
Image
Examples
Embodiment Construction
[0065] refer to figure 1 . The specific steps of the hypersonic vehicle neural network compound learning control method based on the robust design of the present invention are as follows:
[0066] (a) Establish a hypersonic vehicle longitudinal channel dynamics model:
[0067]
[0068]
[0069]
[0070]
[0071]
[0072] The longitudinal channel dynamics model consists of five state variables X=[V,h,α,γ,q] T and two control inputs U=[δ e ,β] T Composition; where, V represents velocity, γ represents track inclination, h represents height, α represents angle of attack, q represents pitch angular velocity, δ e is the rudder deflection angle, β is the throttle valve opening; T, D, L and M yy Represent thrust, drag, lift and pitching torque respectively; m, I yy , μ and r represent the mass, the moment of inertia of the pitch axis, the gravitational coefficient and the distance from the center of the earth;
[0073] The relevant torque and parameters are defin...
PUM
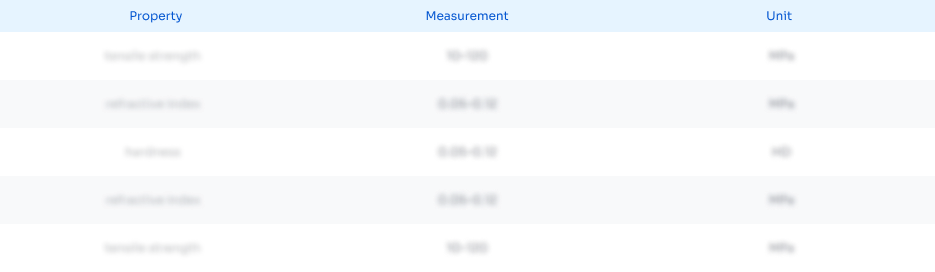
Abstract
Description
Claims
Application Information
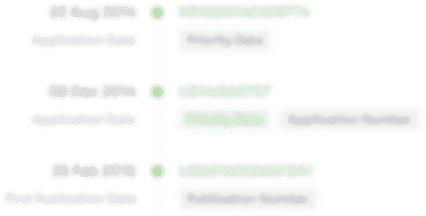
- Generate Ideas
- Intellectual Property
- Life Sciences
- Materials
- Tech Scout
- Unparalleled Data Quality
- Higher Quality Content
- 60% Fewer Hallucinations
Browse by: Latest US Patents, China's latest patents, Technical Efficacy Thesaurus, Application Domain, Technology Topic, Popular Technical Reports.
© 2025 PatSnap. All rights reserved.Legal|Privacy policy|Modern Slavery Act Transparency Statement|Sitemap|About US| Contact US: help@patsnap.com