Geomagnetic field prediction method based on long-short term memory (LSTM) model recurrent neural network
A technology of cyclic neural network and prediction method, which is applied in the field of geomagnetic field prediction based on the long-short-term memory model cyclic neural network, and can solve the problem of low spatial resolution of the spherical harmonic analysis method, increase in the number of spherical harmonic coefficients, and the amount of calculation and storage Increase and other issues, to achieve the effect of enhancing the processing of timing relations, fewer parameters, and simple structure
- Summary
- Abstract
- Description
- Claims
- Application Information
AI Technical Summary
Problems solved by technology
Method used
Image
Examples
Embodiment
[0041] This example provides a method for predicting the geomagnetic field based on the long-short-term memory model recurrent neural network. This method can predict the geomagnetic field of a certain place and has strong real-time performance.
[0042] In this example, the data of the Beijing Ming Tombs Geomagnetic Monitoring Station from August 4, 2017 to August 10, 2017 is selected, and the sampling period is 1 minute. The selected space physical index input includes solar wind temperature, density and velocity, and geomagnetic activity index The sampling period of Dst and Kp is consistent with the sampling period of geomagnetic field data, which is 1 minute. The dataset is a time series with a length of 10080. Among them, the K index is an index used by a single geomagnetic station to describe the intensity of geomagnetic disturbance in every 3 hours of a day, called the three-hour index or magnetic index. The Kp index is the average K value of 13 geomagnetic stations se...
PUM
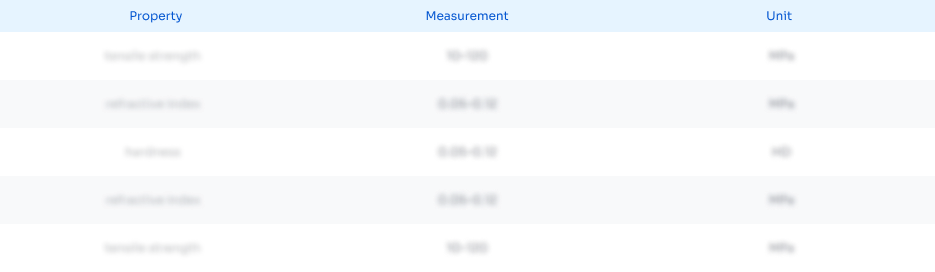
Abstract
Description
Claims
Application Information
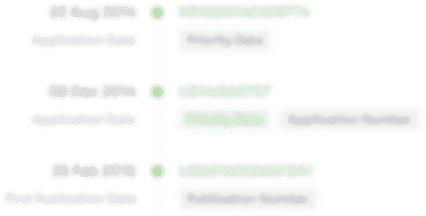
- R&D Engineer
- R&D Manager
- IP Professional
- Industry Leading Data Capabilities
- Powerful AI technology
- Patent DNA Extraction
Browse by: Latest US Patents, China's latest patents, Technical Efficacy Thesaurus, Application Domain, Technology Topic, Popular Technical Reports.
© 2024 PatSnap. All rights reserved.Legal|Privacy policy|Modern Slavery Act Transparency Statement|Sitemap|About US| Contact US: help@patsnap.com