Training method and apparatus of network models, equipment, and storage medium
A network model and training method technology, applied in the field of deep learning, can solve the problems of under-fitting, over-fitting of high-level features of parameter transfer paths, and unsatisfactory processing results, and achieve the effect of high accuracy and thorough training.
- Summary
- Abstract
- Description
- Claims
- Application Information
AI Technical Summary
Problems solved by technology
Method used
Image
Examples
Embodiment 1
[0030] Figure 1a It is a flow chart of a network model training method provided by Embodiment 1 of the present invention. This embodiment can adapt to the situation where the parameter transmission path in the network model is too long and needs to optimize the network model. This method can be provided by the embodiment of the present invention A network model training device is implemented, and the device can be implemented in software and / or hardware. refer to Figure 1a , the method may specifically include the following steps:
[0031] S110. When the first network model reaches the preset update stop condition, determine the first target network model according to the update result of the first network model, and insert after the preset pooling layer in the first target network model The subsequent loss network layer is used to determine the second network model.
[0032] Specifically, during the training process of the first network model, a preset update stop conditio...
Embodiment 2
[0049] Figure 2a It is a flow chart of a method for training a network model provided by Embodiment 2 of the present invention. This embodiment is implemented on the basis of the foregoing embodiments. refer to Figure 2a , the method may specifically include the following steps:
[0050] S210. Input the picture to be trained into the first network model for training, and update the first network model according to the training result.
[0051] Wherein, the picture to be trained is input into the first network model for training, and the first network model is updated according to the training result. Optionally, the pictures to be trained may be pictures in a specific scene, and the specific scene may be a VTM (Video Teller Machine, remote teller machine), member identification in a jewelry store, and the like. Collect face photos in a specific scene, use the camera to collect video pictures, and store them in the computer system through network transmission and data line...
Embodiment 3
[0070] image 3 It is a flow chart of a network model training method provided by Embodiment 3 of the present invention. On the basis of the above embodiments, this embodiment applies the relay loss function and the global loss function to the second The parameters of the network model are updated to obtain the updated second network model" and optimized. refer to image 3 , the method may specifically include the following steps:
[0071] S310. When the first network model reaches the preset update stop condition, determine the first target network model according to the update result of the first network model, and insert it after the preset pooling layer in the first target network model The subsequent loss network layer is used to determine the second network model.
[0072] S320. Determine a relay loss function corresponding to the relay loss network layer according to the second network model and the relay loss network layer.
[0073] S330. Determine a global loss fu...
PUM
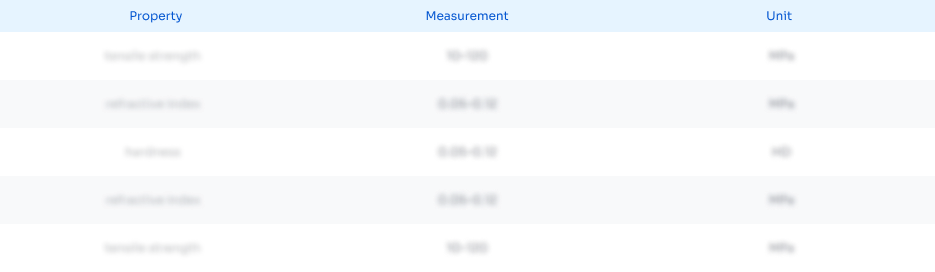
Abstract
Description
Claims
Application Information
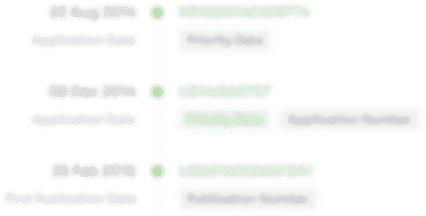
- R&D Engineer
- R&D Manager
- IP Professional
- Industry Leading Data Capabilities
- Powerful AI technology
- Patent DNA Extraction
Browse by: Latest US Patents, China's latest patents, Technical Efficacy Thesaurus, Application Domain, Technology Topic.
© 2024 PatSnap. All rights reserved.Legal|Privacy policy|Modern Slavery Act Transparency Statement|Sitemap