Bearing fault diagnosis method using probabilistic principal component analysis to enhance cyclic bispectrum
A principal component analysis and fault diagnosis technology, which can be used in the testing of mechanical bearings, measuring devices, and mechanical components, etc., and can solve the problem that fault signals are susceptible to noise interference.
- Summary
- Abstract
- Description
- Claims
- Application Information
AI Technical Summary
Problems solved by technology
Method used
Image
Examples
specific Embodiment 1
[0080] Specific embodiment 1: In order to verify the correctness of the bearing fault diagnosis method of probabilistic principal component analysis and enhanced cyclic bispectrum, according to the bearing fault characteristics, a digital simulation model is established:
[0081]
[0082] In formula (20), n(t) is a stationary random signal with zero mean value, h(t) is a series of repetitive pulse shock trains generated by bearing faults, A i is the amplitude of random modulation, T is the impact interval between two adjacent pulses, τ i Indicates the tiny fluctuation of the i-th shock relative to the average fault period T, where i is the number of shocks.
[0083] The response of the bearing to each pulse, defined as:
[0084] h(t)=e -Bt sin 2πf n t (21)
[0085] In formula (21), B is a coefficient determined by bearing resonant frequency, mass coefficient and stress relaxation time, f n is the center frequency of the bearing.
[0086] The severity of bearing fault...
specific Embodiment 2
[0096] Specific embodiment 2: Bearing inner ring fault diagnosis
[0097] Take the fault signal of the inner ring of a real transmission system bearing. The outer ring of the bearing used in the experiment has a fault. 33.4772mm, the theoretical calculation result of the fault characteristic frequency of the rolling bearing inner ring is 197.1Hz. The experimental bearing runs with no load, the speed is 2990r / min, the sampling frequency is 25.6kHz, and the number of sampling points is 327680. In the sampling points, the number of samples m=327660 is selected as the original data for preprocessing, and it is divided into 20 segments, that is, the number of original variables n =20; set the number of pivot variables k=2, then the original data is expressed as X 20×327660 in matrix form.
[0098] Its original signal waveform and spectrum diagram are as follows Figure 6 As shown, the original data signal is also preprocessed by probabilistic principal component analysis and den...
PUM
Property | Measurement | Unit |
---|---|---|
Diameter | aaaaa | aaaaa |
Pitch circle diameter | aaaaa | aaaaa |
Abstract
Description
Claims
Application Information
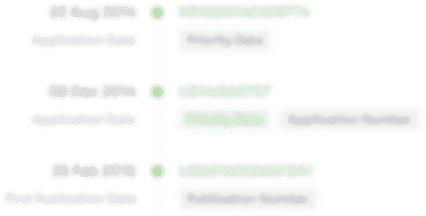
- R&D
- Intellectual Property
- Life Sciences
- Materials
- Tech Scout
- Unparalleled Data Quality
- Higher Quality Content
- 60% Fewer Hallucinations
Browse by: Latest US Patents, China's latest patents, Technical Efficacy Thesaurus, Application Domain, Technology Topic, Popular Technical Reports.
© 2025 PatSnap. All rights reserved.Legal|Privacy policy|Modern Slavery Act Transparency Statement|Sitemap|About US| Contact US: help@patsnap.com