Variable-angle illumination tomography method and device based on deep learning generation network
A deep learning and tomography device technology, applied in neural learning methods, biological neural network models, 2D image generation, etc., can solve problems such as elongation of the optical axis direction, limitation of optical axis tomographic resolution, errors, etc., to achieve Improved resolution accuracy, high tomographic reconstruction capabilities, and high-resolution effects
- Summary
- Abstract
- Description
- Claims
- Application Information
AI Technical Summary
Problems solved by technology
Method used
Image
Examples
Embodiment Construction
[0047] Embodiments of the present invention are described in detail below, examples of which are shown in the drawings, wherein the same or similar reference numerals designate the same or similar elements or elements having the same or similar functions throughout. The embodiments described below by referring to the figures are exemplary and are intended to explain the present invention and should not be construed as limiting the present invention.
[0048] The following describes the variable-angle illumination tomography method and device based on the deep learning generation network according to the embodiments of the present invention with reference to the accompanying drawings. First, the variable-angle illumination layer based on the deep learning generation network proposed according to the embodiments of the present invention will be described with reference to the accompanying drawings. analysis method.
[0049] figure 1 It is a flowchart of a variable-angle illumin...
PUM
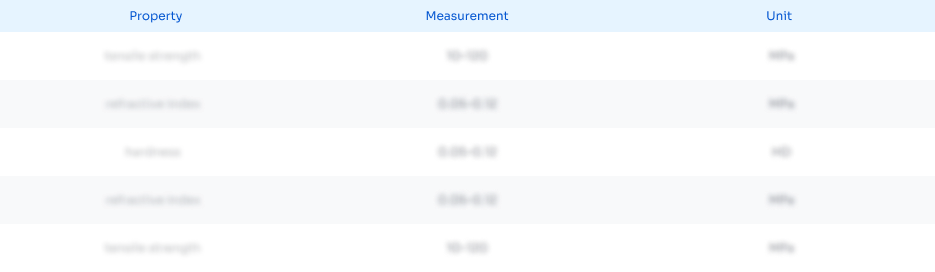
Abstract
Description
Claims
Application Information
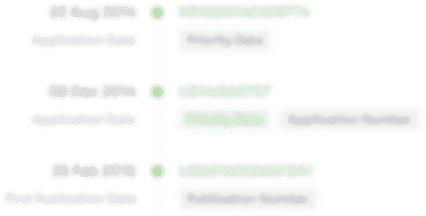
- R&D
- Intellectual Property
- Life Sciences
- Materials
- Tech Scout
- Unparalleled Data Quality
- Higher Quality Content
- 60% Fewer Hallucinations
Browse by: Latest US Patents, China's latest patents, Technical Efficacy Thesaurus, Application Domain, Technology Topic, Popular Technical Reports.
© 2025 PatSnap. All rights reserved.Legal|Privacy policy|Modern Slavery Act Transparency Statement|Sitemap|About US| Contact US: help@patsnap.com