K-SVD learning dictionary based woven fabric texture flaw detection method
A defect detection and dictionary learning technology, applied in the field of image analysis, can solve problems such as large amount of calculation and unstable detection effect
- Summary
- Abstract
- Description
- Claims
- Application Information
AI Technical Summary
Problems solved by technology
Method used
Image
Examples
Embodiment 1
[0082] A woven fabric texture defect detection method based on K-SVD learning dictionary, the specific steps are as follows:
[0083] (1) Image processing;
[0084] First divide the entire woven fabric texture image into n sub-images arranged in rows and columns, and start numbering from 1 to n. The number of each row increases from left to right, and the number of each column increases from top to bottom. Each sub-image has its corresponding number, row number and column number, and then each sub-image is expanded into a column vector, and n column vectors jointly form a test sample image matrix Y, Y=[y 1 ,y 2 ,...y t ,...y n ],y t ∈ R u ,y t The column vector obtained by expanding the sub-image numbered t, t=1,2,...,n,y t =[y lt ,...,y qt ,...,y ut]',y qt for y t The qth element in, q=1,2,...,u, u is y t dimension, the size of the sub-image is 8-64×8-64 pixels;
[0085] (2) Build an initial dictionary D;
[0086] First construct a One-dimensional DCT matrix ...
Embodiment 2
[0138] A kind of woven fabric texture defect detection method based on K-SVD learning dictionary, concrete steps are consistent with embodiment 1, difference is the test sample image selected in step (4), such as Figure 2a As shown, the final defect detection results obtained after the program is completed are as follows Figure 2b Shown, as can be seen from the figure, the detection method of the present invention detects accurately. Run the program code of the embodiment of the present invention 2 many times, select two learning dictionaries y1 and y2 arbitrarily therefrom and implement the regression analysis and obtain the regression model of y=x, i.e. y1=y2, the result is as follows image 3 As shown, it can be seen that the method of the present invention can effectively realize the repeatability of the K-SVD learning dictionary. The results of Examples 1 and 2 show that the learning dictionary algorithm based on K-SVD of the present invention can not only approximate ...
PUM
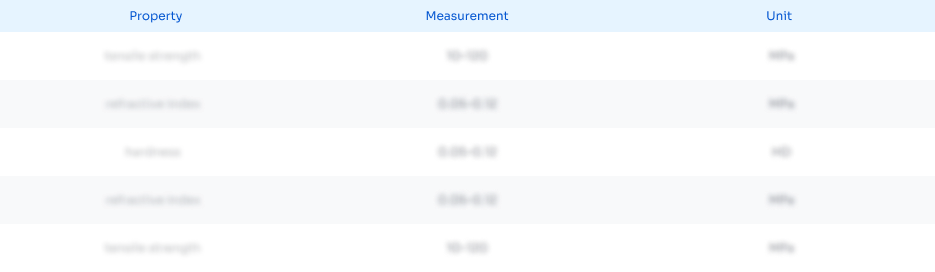
Abstract
Description
Claims
Application Information
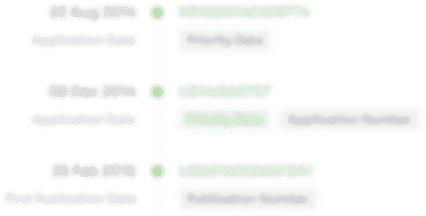
- R&D Engineer
- R&D Manager
- IP Professional
- Industry Leading Data Capabilities
- Powerful AI technology
- Patent DNA Extraction
Browse by: Latest US Patents, China's latest patents, Technical Efficacy Thesaurus, Application Domain, Technology Topic, Popular Technical Reports.
© 2024 PatSnap. All rights reserved.Legal|Privacy policy|Modern Slavery Act Transparency Statement|Sitemap|About US| Contact US: help@patsnap.com