Generation method of convolutional neural networks and expression recognition method
A convolutional neural network and expression recognition technology, applied in the field of image processing, can solve problems such as difficult real-time recognition of expressions, low calculation efficiency, and influence of expressions, and achieve the effects of reducing operation and maintenance costs, improving the accuracy of results, and improving user experience
- Summary
- Abstract
- Description
- Claims
- Application Information
AI Technical Summary
Problems solved by technology
Method used
Image
Examples
Embodiment Construction
[0029] Exemplary embodiments of the present disclosure will be described in more detail below with reference to the accompanying drawings. Although exemplary embodiments of the present disclosure are shown in the drawings, it should be understood that the present disclosure may be embodied in various forms and should not be limited by the embodiments set forth herein. Rather, these embodiments are provided for more thorough understanding of the present disclosure and to fully convey the scope of the present disclosure to those skilled in the art.
[0030] figure 1 is a block diagram of an example computing device 100 . In a basic configuration 102 , computing device 100 typically includes system memory 106 and one or more processors 104 . A memory bus 108 may be used for communication between the processor 104 and the system memory 106 .
[0031] Depending on the desired configuration, processor 104 may be any type of processing including, but not limited to, a microprocess...
PUM
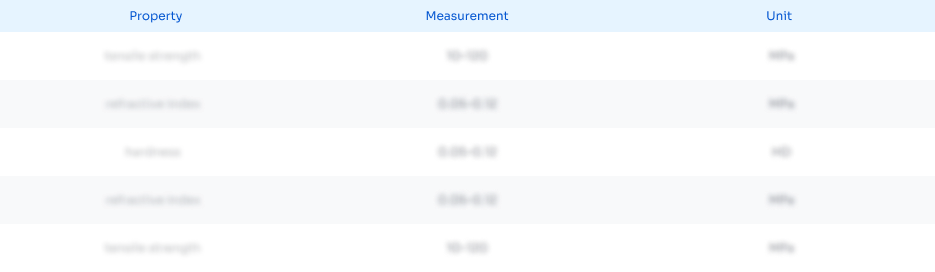
Abstract
Description
Claims
Application Information
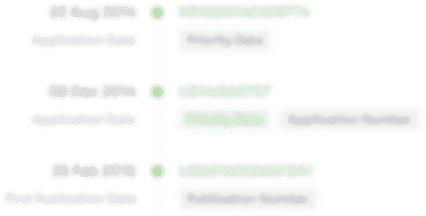
- R&D Engineer
- R&D Manager
- IP Professional
- Industry Leading Data Capabilities
- Powerful AI technology
- Patent DNA Extraction
Browse by: Latest US Patents, China's latest patents, Technical Efficacy Thesaurus, Application Domain, Technology Topic, Popular Technical Reports.
© 2024 PatSnap. All rights reserved.Legal|Privacy policy|Modern Slavery Act Transparency Statement|Sitemap|About US| Contact US: help@patsnap.com